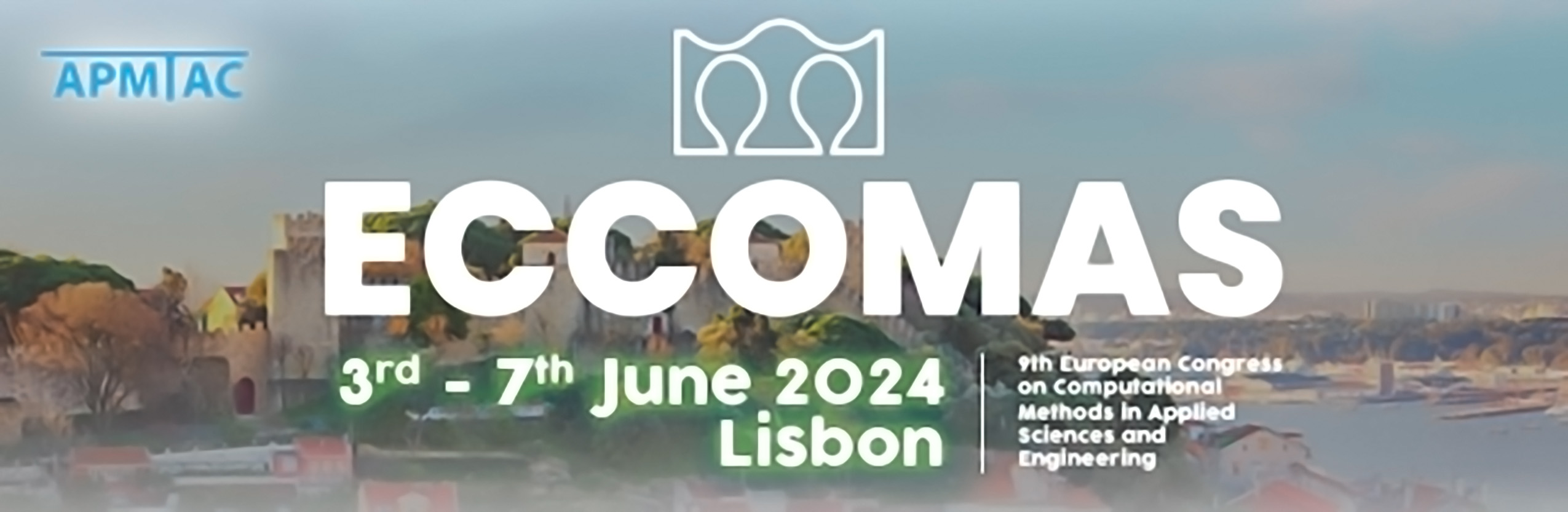
Regularization Techniques for Data-driven Reduced Order Models
Please login to view abstract download link
The focus of the present contribution is related to Galerkin-Reduced Order Models (G- ROMs) applied to the study of advection-dominated flows. In order to ensure a low ROM computational cost, we build the model with relatively few basis functions, namely an under-resolved or marginally-resolved regime is considered. In the above-mentioned regimes, the standard G-ROMs generally yields inaccurate results and spurious oscillations, even though the number of modes is enough to capture the dynamics of the system. To increase the accuracy of standard G-ROMs, a variety of machine learning (ML)-based closure strategies have been studied to re-integrate the contribution of the neglected basis functions. Moreover, different regularization techniques have been investigated in the last few years to alleviate spurious oscillations at the reduced order level (Regularized-ROMs), such as Evolve-Filter-Relax ROMs (EFR-ROMs) and Leray-ROMs. In this contribution, we propose a regularization technique able to smooth out the reduced order solution and to increase the accuracy of the standard G-ROM. The data-driven regularization operator is built making use of machine learning tools and is tested on well-known test cases in literature, such as the two-dimensional turbulent flow past a cylinder.