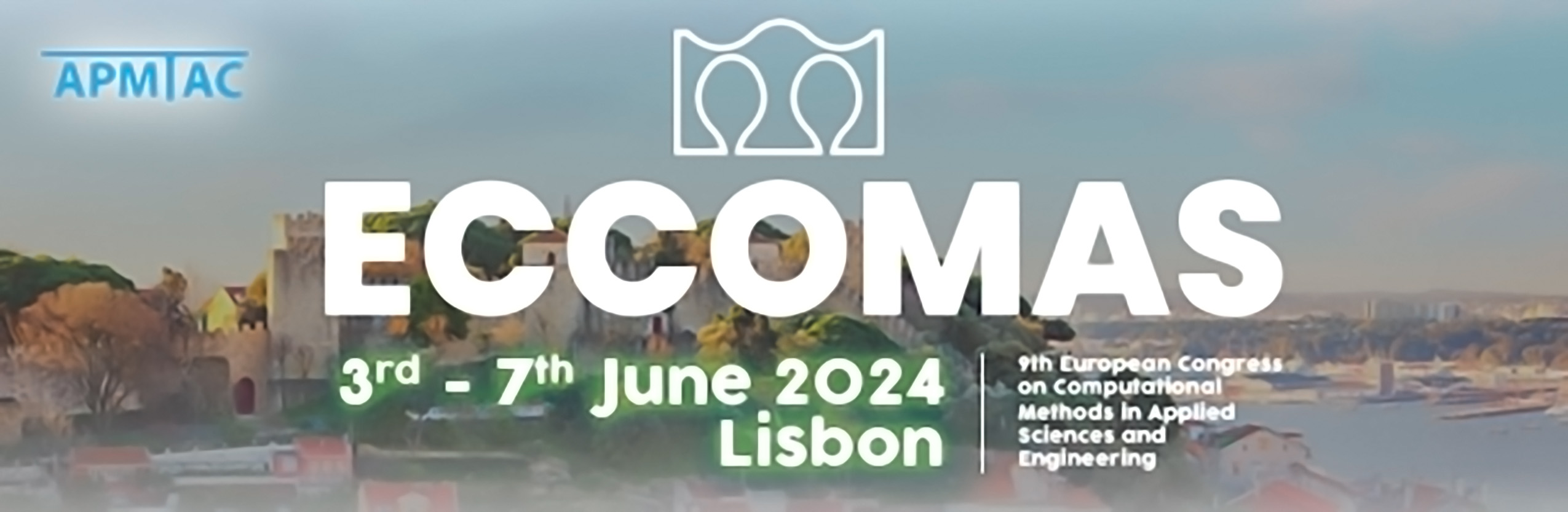
Machine Learning for the Inverse Design of Architected Materials
Please login to view abstract download link
Identifying optimal structural designs that furnish architected materials with target effective, homogenized mechanical properties is a common challenges in solid and structural mechanics. We will survey the application of machine learning (ML) to this challenges and present opportunities for bypassing expensive computational modeling and optimization through the use of ML-based models. Specifically, we will present the use of Variational Autoencoders combined with a graph representation of trusses to predict and optimize the homogenized anisotropic stiffness and the homogenized nonlinear stress-strain response of general periodic truss lattices. In addition, we show how video diffusion models can predict optimal cellular structures with as-designed homogenized nonlinear stress-strain response. In this case, the ML-based model not only efficiently identifies optimal designs with target properties but also predicts the full-field stress and strain distribution inside the sample, thus bypassing the optimization step as well as the full-field finite element simulation. In both cases, we demonstrate that the generative ML models generalize well outside the training set, meaning that new designs can be predicted with properties not encountered during training the neural networks.