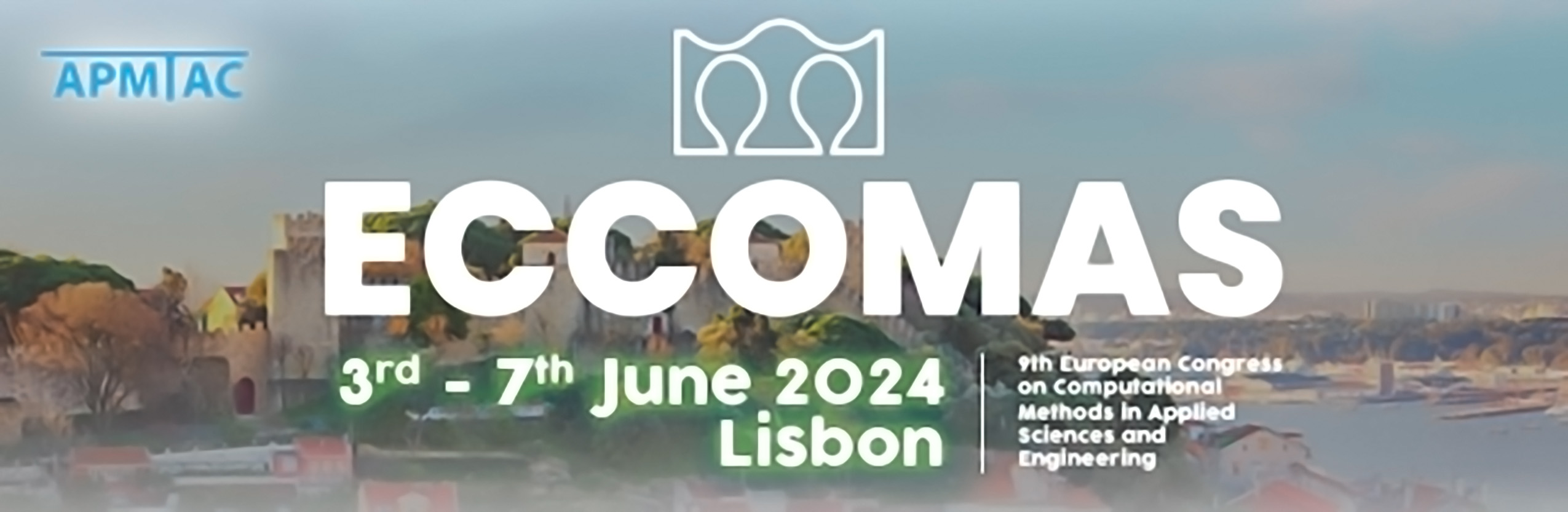
Multiple limit-states reliability analysis for continuous reinforced concrete beams
Please login to view abstract download link
In the context of uncertainty propagation, conducting efficient structural reliability analysis is crucial for the design and analysis of computationally expensive engineering problems, such as the design of reinforced concrete rail bridges. Reliability analysis involves calculating the failure probabilities of an engineering system with respect to one or multiple limit-state functions. To address this, various methods, including analytical approaches, sampling techniques, and surrogate-based algorithms can be found in the existing literature. To avoid substantial calls for computationally expensive FEM models of complex engineering systems, active learning surrogate-based techniques can be used nowadays to deal with such problems. To perform active learning reliability analysis, four steps should be done starting with surrogate model development, followed by failure probabilities estimation, applying then a learning function to enrich the surrogate model, to checking finally the convergence of the method. The objective of the presented work is to conduct a reliability analysis involving multiple limit-states for a continuous reinforced concrete beam. The limit states considered are the admissible deflection, both flexural and shear strengths, and the admissible stress in the steel rebars for the cracking control check. The analysis is performed using a kriging surrogate based on a limited number of simulations of a multi-fiber non-linear FEA RC beam model implemented into Cast3M software, in combination with subset simulation, deviation number U as a learning function, and a combined stopping criterion. A comparative performance assessment is also conducted on different active learning algorithms generated by substituting the learning function alone or both the surrogate and the learning function. The number of iterations required by the model to converge is the main criterion for the performance assessment.