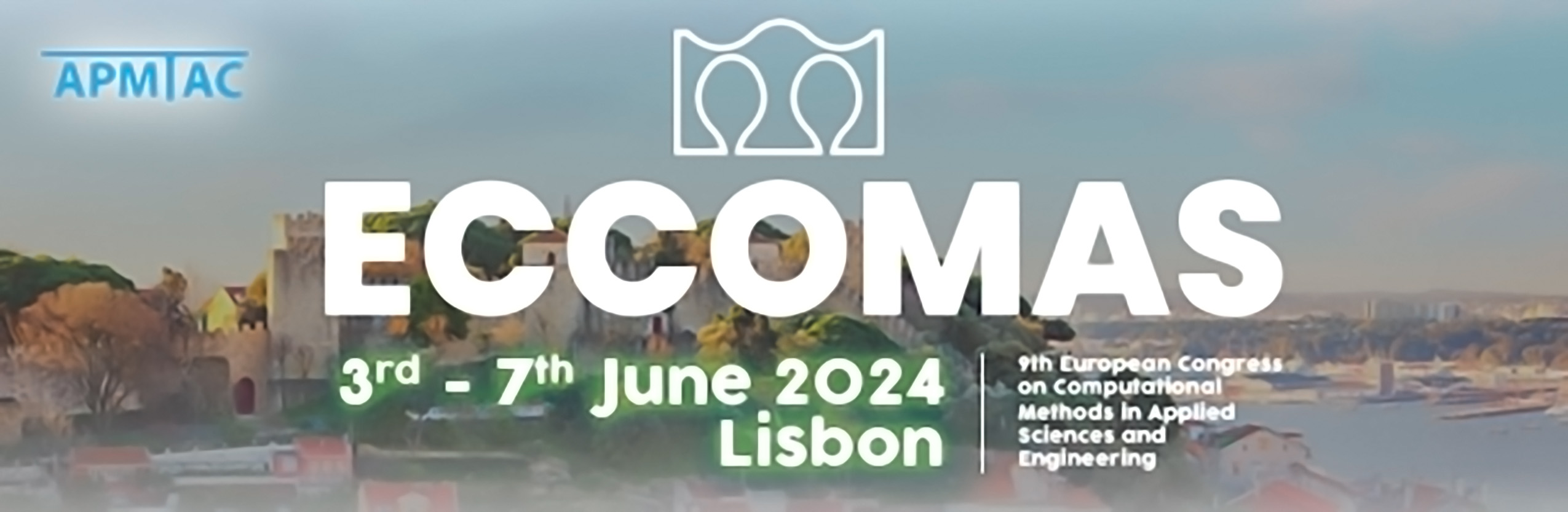
Application of machine learning to accelerate explicit time integration in structural dynamics
Please login to view abstract download link
Stability of explicit time integration in structural dynamics, e.g.~using finite elements, is limited by a critical time step. However, computing it exactly by solving the corresponding eigenvalue problem is too expensive. Therefore, there are several approaches to estimate the critical time step, many of them through an estimation on the element level. A popular and inexpensive approach is to estimate the critical time step via heuristic formulas based on a characteristic element length. The estimate is obtained by dividing it by the wave speed. For instance, the characteristic length of a 2D solid element can be calculated by the element area divided by its longest side or its longest diagonal. However, the resulting estimates are usually non-conservative, which necessitates a safety factor to prevent instabilities. For more complex elements the estimate can be even less accurate, as many influencing factors may be neglected when heuristic formulas are used. More accurate time step estimators can be derived by utilizing data sets of relevant element shapes within a data-integrated machine learning approach. This contribution presents the basic concept and extends it to a broader range of finite elements, showcasing the generality and reliability of the method. It is an example for applying methods of machine learning to computational mechanics in a way that exploits the efficiency of neural networks while avoiding the downside of relying on black-box approaches.