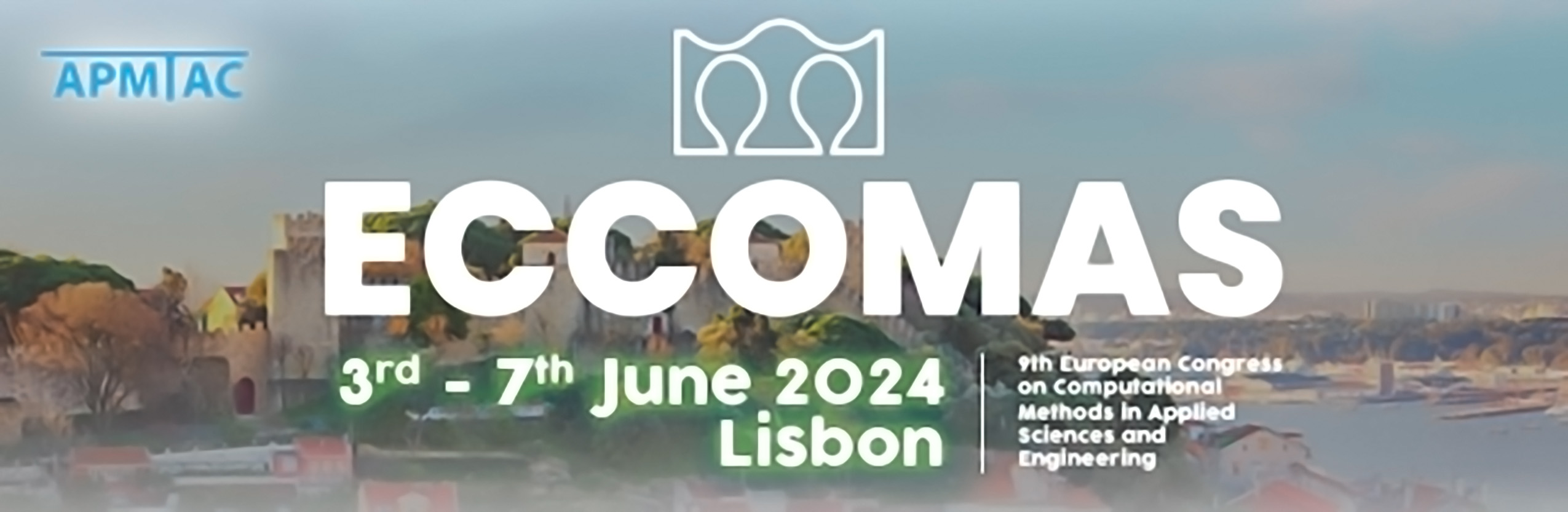
Enhancing Constraint-handling in Crash through Bayesian Optimization with Constraint-driven Trust Regions
Please login to view abstract download link
Gradient-free optimization algorithms face challenges in locating optimal solutions when working under black-box constraints, meaning that the mathematical expressions of the constraints are unknown. This is evident when the feasibility areas are non-convex and small, hence difficult to find through sampling. One field affected is crashworthiness optimization in the context of engineering design. In this field, Bayesian Optimization (BO) shows strong potential in optimizing the crash performance of components [2]. However, this is not true when incorporating constraints that render most of the domain infeasible. Constrained BO algorithms typically train global surrogates on the constraints. Then, they define a probability of feasibility to guide the optimization procedure [3]. Other algorithms restrict the surrogates of the constraints to evolving regions of interest (trust regions) [1]. These regions are defined at each iteration according to the current optimum. In this study, we leverage the concept of trust regions but introduce a novel scheme to guide the optimization towards the feasible areas of the search space, addressing the challenge of small feasible areas. To achieve this, we use the surrogate of the constraints to define the regions of interest. At each iteration, we confine the search for the next candidate solution to a region with a high probability of feasibility. We validate our method using analytical benchmarks and a practical crashworthiness test. We compare its performance with other state-of-the-art gradient-free algorithms for constrained optimization. We show that basing the definition of the trust regions on the predicted information for the constraints helps overcome the limitations associated with small feasible areas. References [1] Eriksson D, Poloczek M. Scalable constrained Bayesian optimization. InInternational Conference on Artificial Intelligence and Statistics 2021 Mar 18 (pp. 730-738). PMLR. [2] Raponi E, Bujny M, Olhofer M, Aulig N, Boria S, Duddeck F. Kriging-assisted topology optimization of crash structures. Computer Methods in Applied Mechanics and Engineering. 2019 May 1;348:730-52. [3] Ungredda J, Branke J. Bayesian optimisation for constrained problems. arXiv preprint arXiv:2105.13245. 2021 May 27.