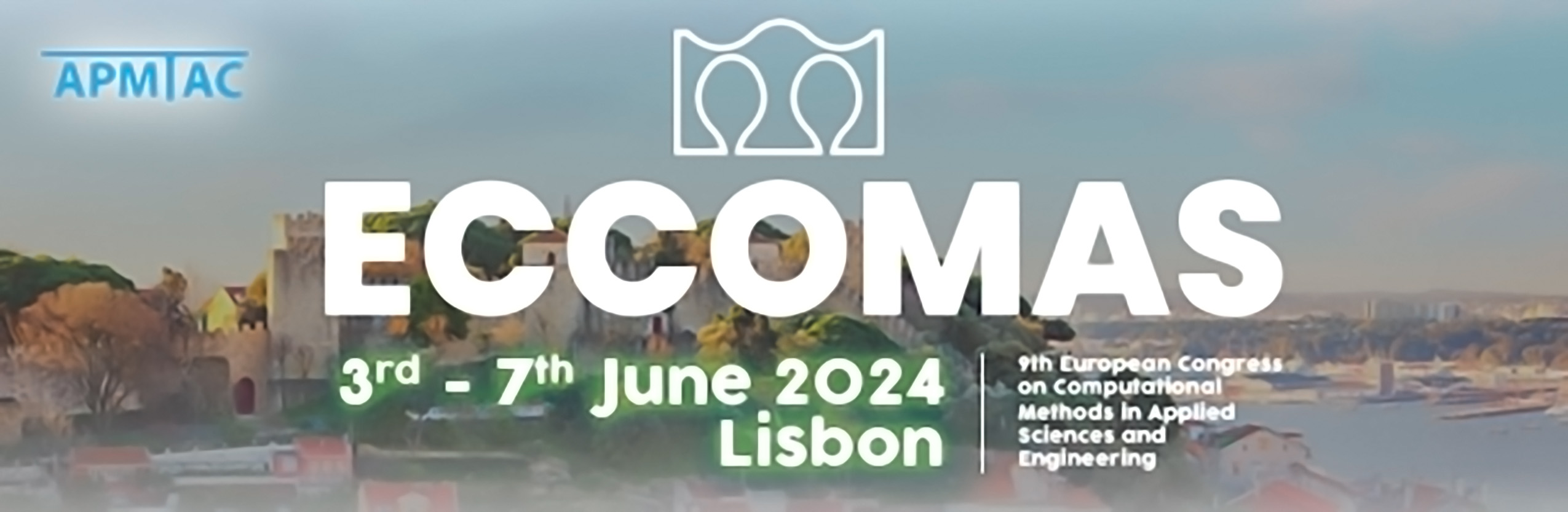
An automated dual-stage approach for constitutive modeling of hyperelastic solids
Please login to view abstract download link
For materials exhibiting complex nonlinear elastic or inelastic behavior, the formulation and calibration of constitutive models remain challenging tasks. Accordingly, in the field of computational mechanics, novel techniques -- often referred to as data-driven or data-based methods -- have gained popularity in recent years. These methods, however, require an extensive amount of data, typically stresses and strains for solid mechanics problems. In this contribution, we present a consistent dual-stage approach for the automated calibration of hyperelastic constitutive models, which only requires experimentally measurable data. In the first step of our approach, data-driven identification (DDI) is applied to determine tuples consisting of stress and strain states [1]. By specifying only the displacement field and the applied boundary conditions -- which may be determined using full-field measurement techniques like digital image correlation (DIC) -- this method enables to identify these data. A physics-augmented neural network (PANN) is calibrated using the data in the second step of the suggested framework [2]. In addition to being extremely flexible, the introduced model fulfills all common conditions of hyperelasticity in a hard way. Moreover, the PANN model can be easily implemented into a finite element (FE) code. We demonstrate the applicability of our approach by several descriptive examples. Therefore, a reference constitutive model is used to generate two-dimensional synthetic data exemplarily. The calibrated PANN is then applied in three-dimensional FE simulations, where the solution is compared to the reference model. [1] Leygue, A., Coret, M., Réthoré, J., Stainier, L. and Verron, E., Data-based derivation of material response, Computer Methods in Applied Mechanics and Engineering 331 (2018). [2] Linden, L., Klein, D. K., Kalina, K. A., Brummund, J., Weeger, O. and Kästner, M., Neural networks meet hyperelasticity: A guide to enforcing physics, Journal of the Mechanics and Physics of Solids 179 (2023).