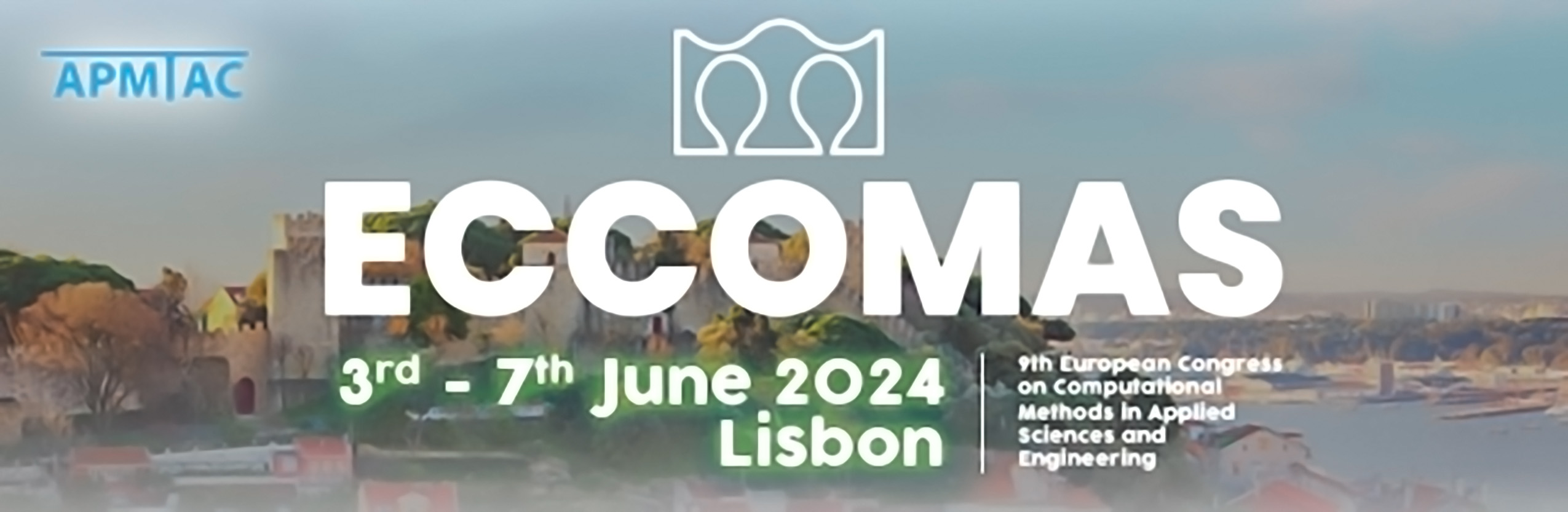
Characterization of Discrete Adjoint Solvers in SU2 for Aerodynamic Design Optimization
Please login to view abstract download link
Aerodynamic design involves solving intricate equations encompassing diverse physical phenomena like shockwaves, turbulence, and heat transfer. Recent advances in computational techniques, high-performance computing, and efficient algorithms have significantly influenced the role of CFD in systematically addressing engineering trade-offs and leveraging beneficial interactions among design variables and constraints, accelerating the generation of improved designs. By formulating design challenges as mathematical optimization problems, it becomes feasible to systematically resolve engineering trade-offs and exploit beneficial interactions among design variables and constraints in large-scale aerodynamic shape optimization (ASO) frameworks. However, the accuracy and computational cost of gradient calculations are crucial, as they can be potential bottlenecks in the design optimization workflow. This work aims to characterize the performance of two different discrete adjoint solvers (1) fixed-point and 2) residuals-based approach) for efficient sensitivity analysis within the SU2 framework. The fixed-point formulation offers advantages such as not requiring factorization and solving of the exact Jacobian matrix, using the same iterative solver for both primal and adjoint problems, and its duality preserving property. However, the convergence of these methods is linear. There has been recent implementation of a new residual-based discrete adjoint solver in SU2, which computes and store previously unavailable partial derivatives of the adjoint system explicitly. This implementation modifies the recording, seeding, and extracting of the automatic differentiation tape to solve the adjoint problem slightly differently, which provides unprecedented access to sensitivity information for aerodynamic design. The SU2 code, uniquely supporting both fixed-point and residuals-based formulations, enables a detailed characterization of the two approaches in terms of adjoint solver accuracy, convergence, and stability.