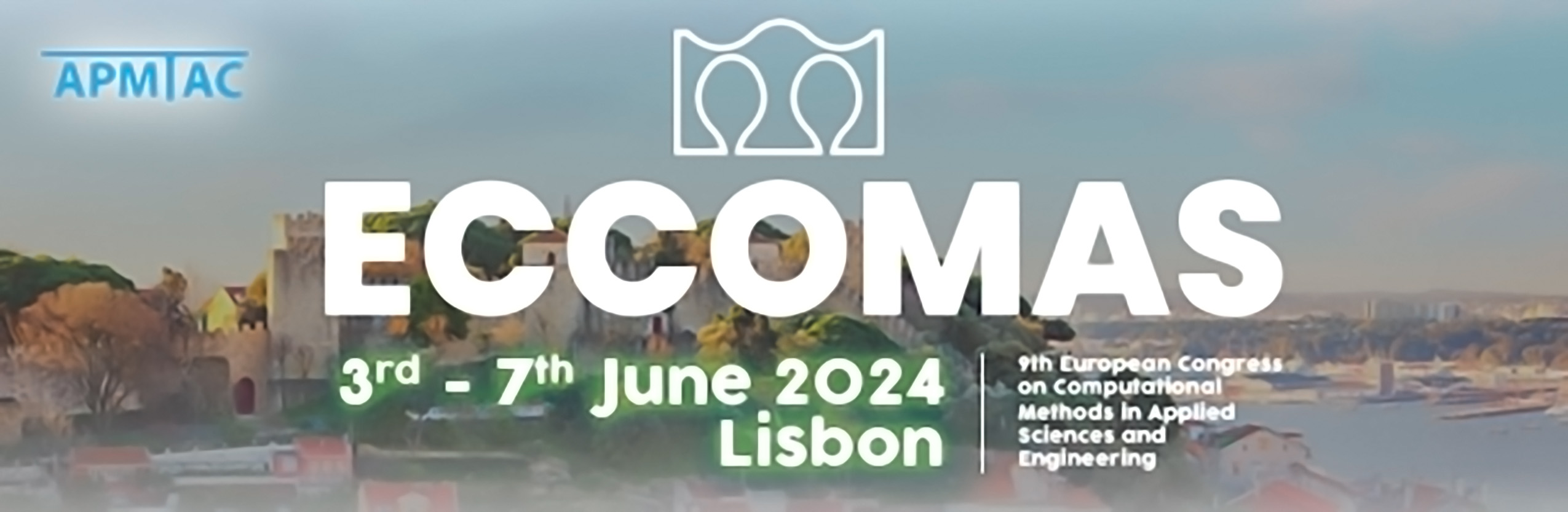
On the Tuning of Patient-Specific Boundary Conditions in Coronary Artery Flow Simulations
Please login to view abstract download link
Introduction. Computational fluid dynamics (CFD) allows non-invasive estimation of fractional flow reserve (FFR) in coronary artery, to assess stenosis severity. However, a reliable result requires to assign realistic boundary conditions (BCs), tuned to patient-specific clinical parameters often unavailable such as coronary flow and mean aortic pressure (MAP) in hyeperemia. While many tuning frameworks are described in the literature, the computed BCs generally requires additional adjustment steps to match patient clinical data [1, 2]. We implemented a framework for assigning BCs based on imaging and baseline parameters only and we compared 4 different, gradually more sophisticated, BCs couplings. Methods. CT-scans of 102 patients for which FFR was invasively acquired. Baseline clinical parameters including heart rate (HR) and systolic/diastolic pressure (SP/DP) were available for each patient. The lumen of coronary arteries and the myocardial wall were segmented from CT. Resting coronary flow was estimated as Q_r=M_myo×8[7×10^(-3) (HR⋅SP)-0.4] [3], where M_myo is the mass of the myocardial wall. Resting coronary resistance was computed as R_r=MAP/Q_r. Hyperemic values were set as in Ref [3]. The BCs couplings summed up in Table I were simulated in SimVascular. At the inlet of coronary arteries, the flow rate was prescribed, while at each coronary outlet a LPM model was prescribed. Resistance and compliance when present, were split in each branch based on Murray’s law. Flow rate was iteratively adjusted until the correct mean aortic pressure of the patient was achieved. Table I. Summary of the simulated cases with average cost per simulation and relative error ε(FFR) between the computed FFR for each case and the invasive measurement. Flow condition Inlet Outlet Average Cost* ε(FFR) Case 1 Transient Flow waveform RCRCR model 6-8 hours 3.9% Case 2 Steady Flow rate R 30-60 min 4.1% Case 3 Steady Flow rate Traction-free 20 min 12.8% Case 4 Steady Flow rate Zero pressure 20 min >20% *Simulated on 40 cores on a server Intel® Xeon® CPU X5670 2.93 GHz 192 Gb RAM Conclusions. Steady simulations, when coupled with resistance LPM proved efficient prediction of the FFR value. This approach performed comparably to more complex transient simulations, but with a significantly lower computational cost, yielding FFR predictions that differed by less than 1%. Conversely, less sophisticated simulations that neglected the patient's microcirculation resistance failed to ac