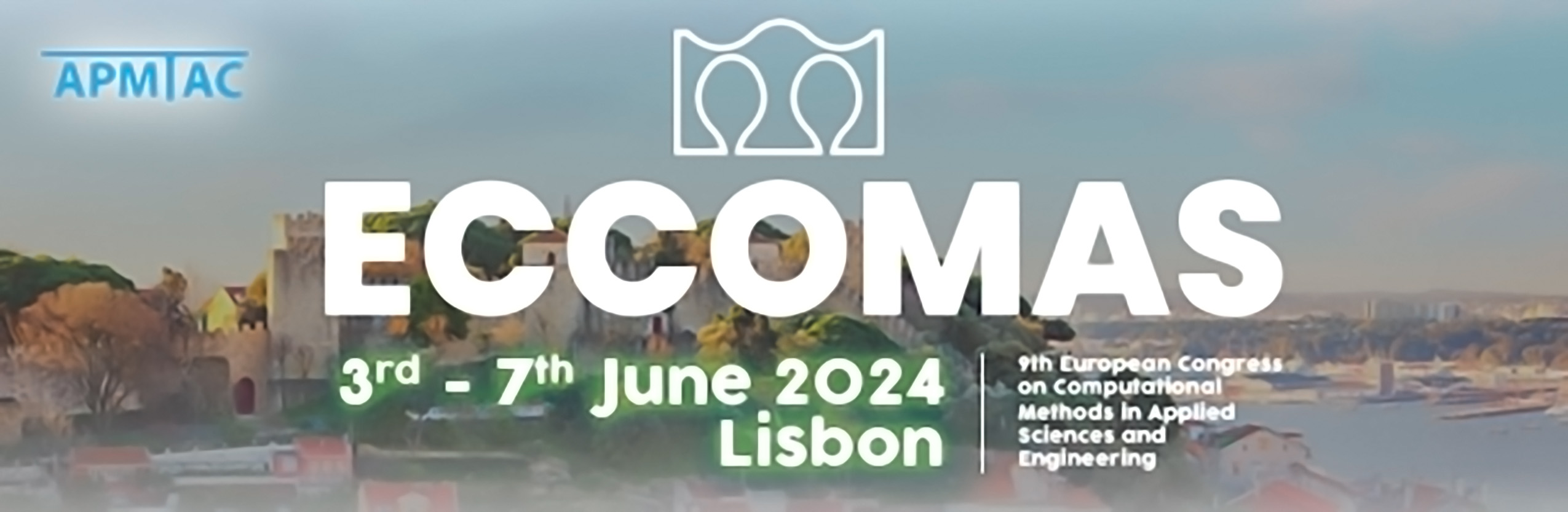
Evaluating Forecast Divergence in Fluid Dynamics: A Metric for Autoregressive Models in the Absence of True Solution Data
Please login to view abstract download link
In this work, we introduce a novel metric designed to quantify the error committed by predictions from an autoregressive forecasting model, in the absence of knowledge of the actual solution. This metric provides a means to determine the appropriate point at which to cease predictions, ensuring that the forecast does not diverge significantly from the true dynamics of the physical system under consideration. The proposed metric was tested on the challenging task of forecasting the dynamics of a turbulent flow at a Reynolds number of Re = 2600, where it demonstrated a high degree of similarity with the actual prediction errors, effectively capturing the divergence between the predicted and true flow dynamics.