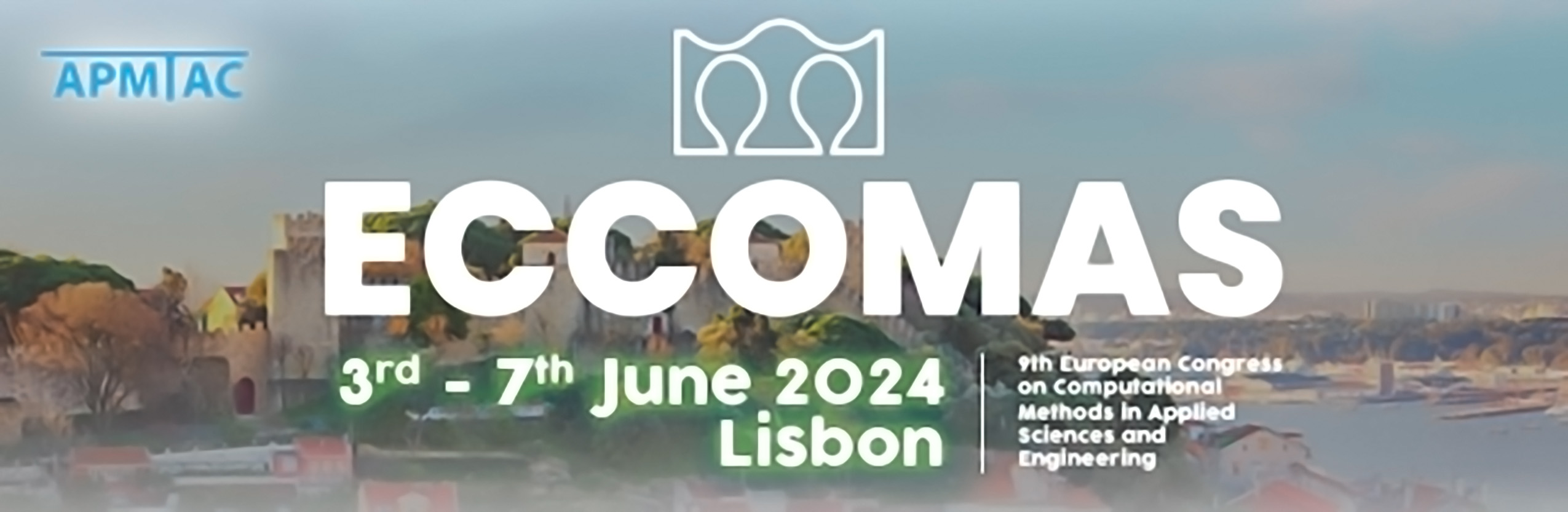
Exploring Nonlinear Preconditioning for Quasi-Newton Methods
Please login to view abstract download link
Linear preconditioning strategies have been used to expedite the convergence of iterative methods and enhance the computational efficiency of many numerical simulations. The standard linear preconditioners have shown remarkable efficacy in accelerating the convergence of iterative solvers. However, in the context of nonlinear systems, which are ubiquitous in various scientific and engineering domains, preconditioning poses significant challenges. Linear preconditioning methods are often ineffective in capturing the intricate nonlinearities inherent in these systems, which limits their effectiveness in improving the efficiency of the overall simulation process. We propose a Nonlinear Restricted Additive Schwarz (NRAS) preconditioning to enhance the convergence rate of limited memory quasi-Newton (QN) methods. This study will consider two distinct strategies: left-preconditioning and right-preconditioning. The standard gradients and Hessians are transformed into their preconditioned counterparts by incorporating nonlinear preconditioning. Consequently, the conventional secant pairs cannot adequately approximate the preconditioned Hessians. We will discuss the methodology for constructing suitable secant pairs within the framework of preconditioned QN. Finally, we will demonstrate the efficiency of the nonlinearly preconditioned QN methods through several numerical experiments.