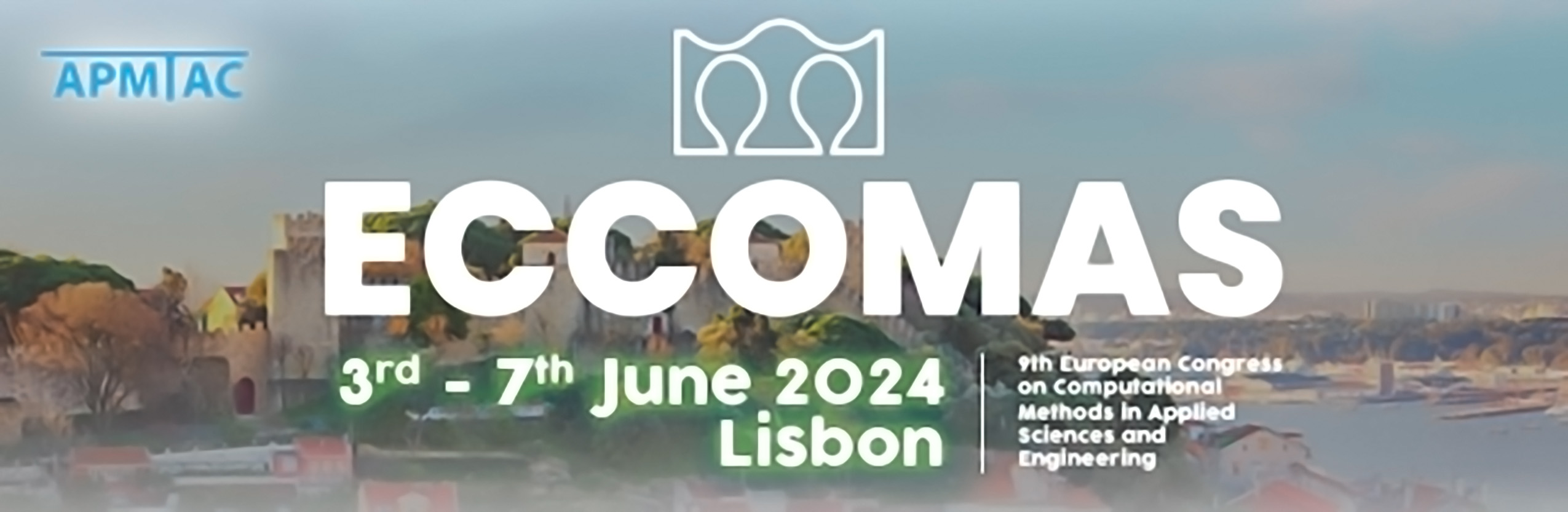
Surrogate-Based Bayesian Updating of Finite Element Solutions for State Monitoring
Please login to view abstract download link
Quantifying parameter uncertainties and model discrepancies is a crucial step towards building faithful digital representations of real-world structures and machines. The statistical Finite Element method (statFEM) [1] has been recently introduced as a candidate for digital twins, endowed with a probabilistic description of model uncertainties. Based on Bayesian principles, statFEM posits a finite element-based prior, which is updated with sparse sensor data. However, there are several challenges associated with a Bayesian updating framework of large-scale finite element models. Among them are the high computational cost of uncertainty propagation, the handling of non-Gaussian distributions induced by nonlinear relationships between model inputs and outputs and dense covariance matrices and their inverses, which appear in posterior formulations. In this talk, we will address statFEM formulations for static and dynamics problems in computational mechanics and focus on surrogate modeling to reduce the computational effort. Surrogates can be employed to cheaply approximate FEM-priors, at the same time, the data model must account for any surrogate error to avoid suboptimal estimation efficiency. We consider both reduced order models and Polynomial Chaos-based surrogates and illustrate the approach with examples, ranging from hyperelastic materials [2] to wave-type problems. We also discuss strategies to condition non-Gaussian densities and to estimate the associated surrogate errors. [1] Girolami, M., Febrianto, E., Yin, G., & Cirak, F. (2021). The statistical finite element method (statFEM) for coherent synthesis of observation data and model predictions. Computer Methods in Applied Mechanics and Engineering, 375, 113533. [2] Narouie, V. B., Wessels, H., & Römer, U. (2023). Inferring displacement fields from sparse measurements using the statistical finite element method. Mechanical Systems and Signal Processing, 200, 110574.