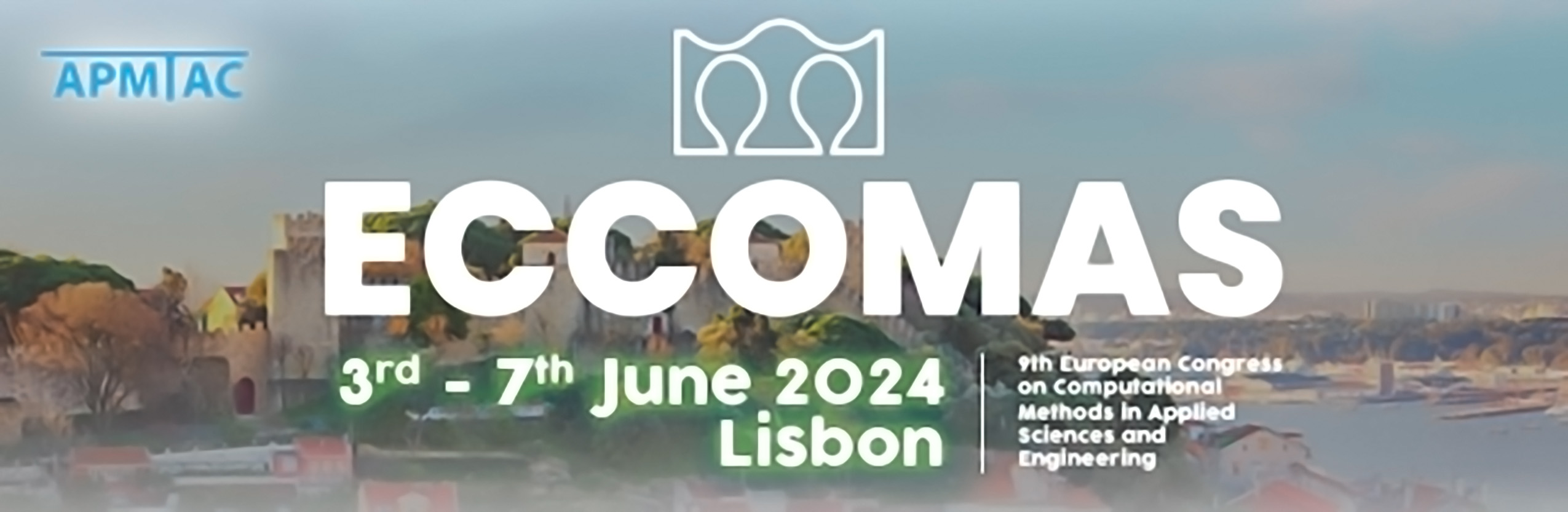
Advances in modeling relative permeability curves under salinity effects using artificial neural networks
Please login to view abstract download link
Artificial neural networks (ANN) have been employed for predicting changes in relative permeability curves due to variations in the salinity of injection water [1,2]. In recent work [3], the incorporation of real data and optimization of network hyperparameters was implemented; however, the models obtained still tended to overestimate the results for the endpoints of the relative permeability curves. Here, we propose modifications to the ANN structure to address this issue, aiming for a better utilization of the available dataset. The ANN consisted of a multilayer feedforward structure with backpropagation, consisting of one network for predicting normalized coordinate points of relative permeability curves, and a second network for predicting the endpoints, the latter utilizing only experimental data without prior normalization. The dual networks were trained for both sandstone and carbonate samples, and hyperparameter optimization was conducted for each model. Previous results suggest an improvement in the accuracy of the models, with a training mean squared error (MSE) on the order of 1e-3 for all models and a more consistent prediction of endpoints. The generated models are suitable for application in modeling relative permeability curves in engineered water injection (EWI) projects, and the methodology can be applied to other multivariate regression problems as well.