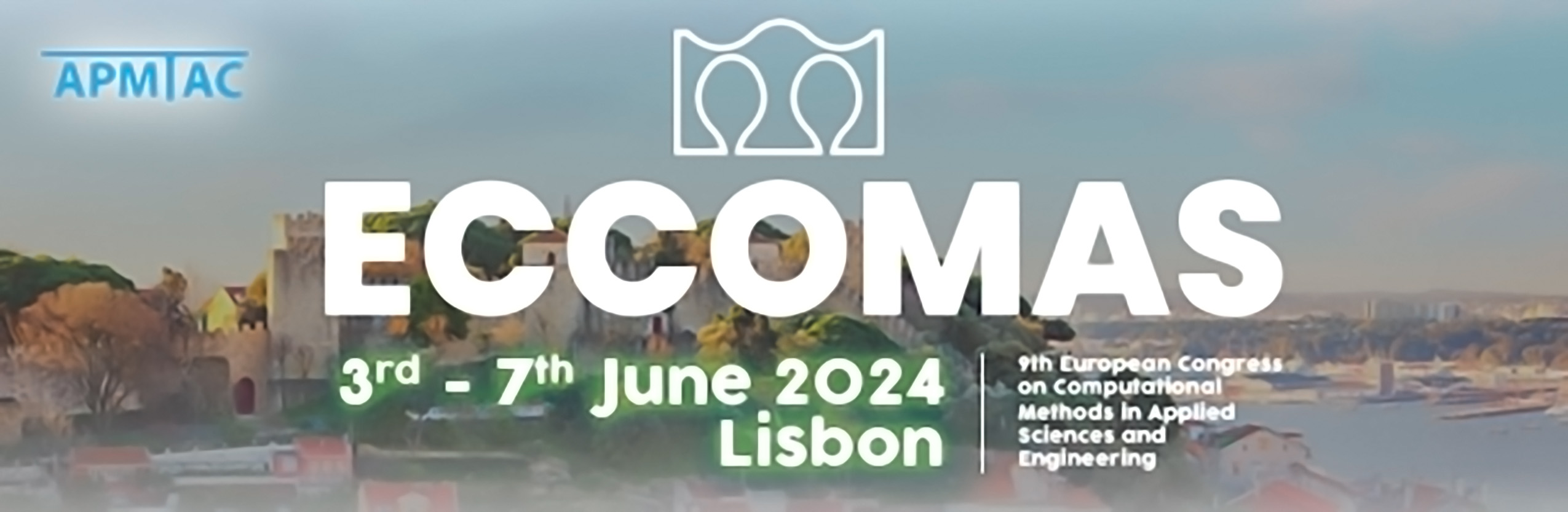
An Efficient Localized Model Order Reduction Framework for the Shape Optimization of Additively Manufactured Lattice Structures
Please login to view abstract download link
A common engineering practice is to optimize the geometry of a structure by an iterative process, in which an objective function is minimized by systematically choosing the value of design variables and computing the value of the objective function many times. However, regarding multiscale problems, this direct numerical approach is not feasible for a number of reasons. The model has to take into account both the structural and unit cell (UC) scale, because the objective function to be minimized is a global quantity, while the parameters are related to the shape or material parameters of the UC. In the absence of a clear separation of scales, the direct resolution of both scales in the numerical model leads to a significant increase in computational cost, which makes it impossible to repeatedly evaluate the model during the optimization process. The main goal of this contribution is to overcome the aforementioned limitations and develop a new efficient computational framework for the optimal design of lattice structures. To this end, parametric MOR is combined with DD methods. The change in geometry of each UC within the lattice is modelled by introducing a transformation that maps the reference to the physical domain. In offline simulations, suitable localized approximation spaces, that account for the change in geometry of the UC and its neighbours, are constructed using the concept of oversampling and random sampling. These are then integrated into a dual DD framework to derive a robust and scalable solver, i.e. a fast-to-evaluate reduced order model (ROM). The ROM is validated on the example of optimizing a concrete arc or pre-stressed multi beam structure minimizing its mass.