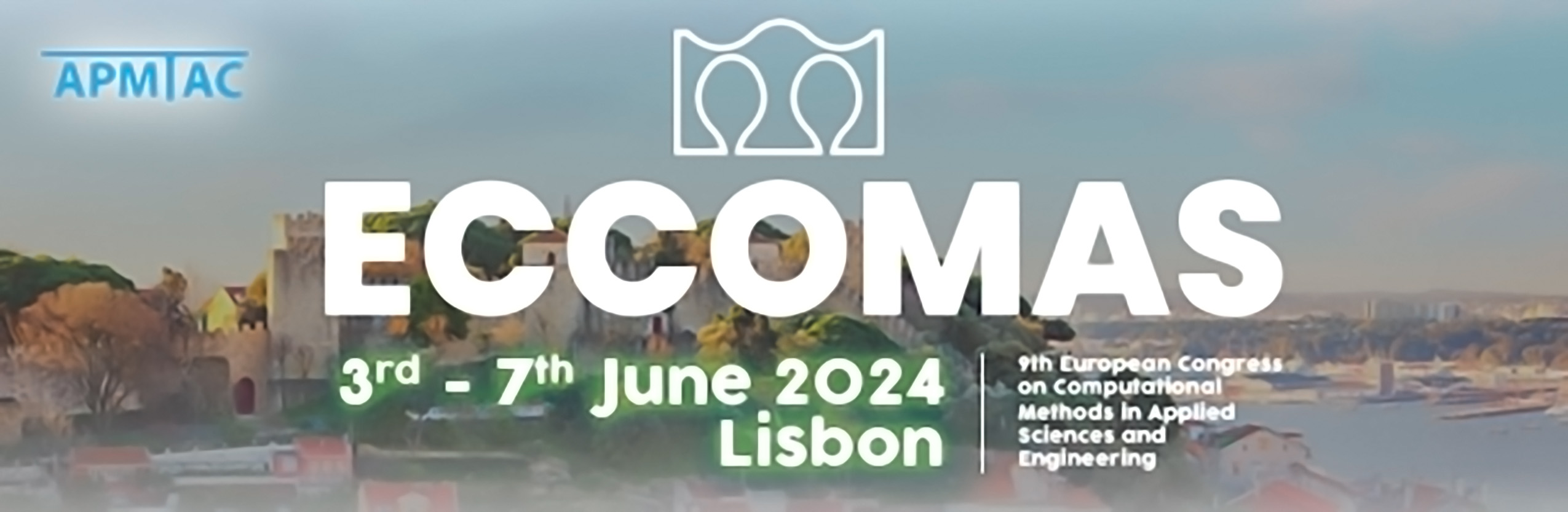
Efficient Gradient-Free Topology Optimization through Latent Space Representation
Please login to view abstract download link
Gradient-free optimizers allow for tackling problems regardless of the smoothness or differentiability of the objective. However, in the past, they received strong criticism in the topology optimization community, due to their increased costs as compared to gradient-based approaches. In our work, we show that these issues can be to a large extent alleviated when optimizing the designs in latent space, opening a new path for optimizing problems when gradient information is not readily available. We train a variational autoencoder and propose to use its latent space for optimizing designs without gradients. Through extensive benchmarking experiments, we show that the reparameterization to a latent space consistently speeds up the optimization by approximately 2 orders of magnitude as compared to the standard gradient-free approaches. Furthermore, we show that the acceleration can be achieved even when the model is employed (without retraining) to optimize problems significantly different from those seen in the training -i.e. with different objectives and physics. We conclude that with our method, gradient-free optimization becomes a feasible approach for topology optimization when gradients are hard to obtain.