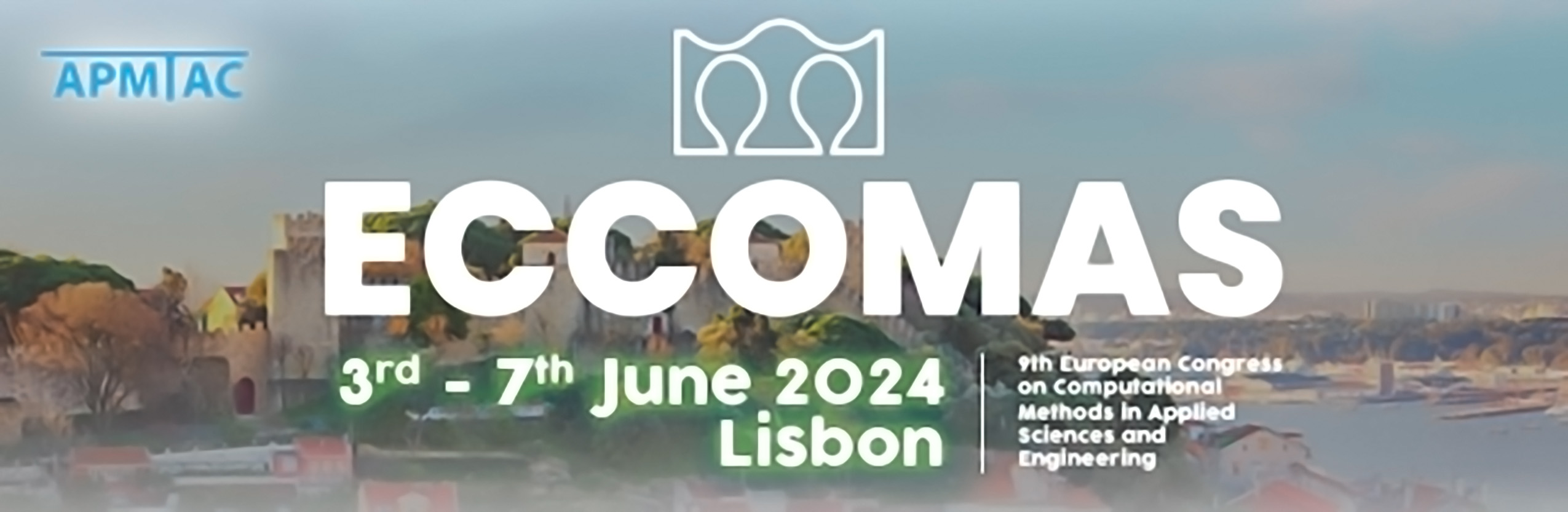
Markov Chain Monte Carlo on Matrix Manifolds for Probabilistic Model Order Reduction
Please login to view abstract download link
The purpose of Model Order Reduction (MOR) is to identify patterns and use them to decrease the number of dimensions of a problem as much as possible. Although often overlooked, it is important to quantify the uncertainty (UQ) associated with the reduction to trust the predictions made by the model. At the same time, incorporating domain knowledge into the UQ process is crucial, especially if data is sparse or noisy, which motivates the use of Bayesian statistics. This work aims to extend classical tools in model order reduction as well as results in the field of optimisation on Riemannian manifolds [1] to the probabilistic case, within a Bayesian framework. We present a method to sample from any given distribution defined on various matrix manifolds. In fact, matrix manifolds, in particular the Grassmann and the Stiefel manifold, are used in model order reduction whenever linear subspaces of appropriate dimensions and orthogonality properties are sought. We present an adaptation of the Metropolis Adjusted Langevin Algorithm (MALA) that allows to draw samples from a posterior probability distribution of a random matrix defined on a matrix manifold. In this way, not only the matrix that maximises the posterior is found, but also the uncertainty around it. This also allows to propagate the uncertainty on the original unreduced problem so to quantify the truncation variance. In case the reduced dimension is not known a priori, we propose an adaptation of the Reversible Jumps Markov chain Monte Carlo method, which allows to navigate manifolds of different dimensions and identify the best reduced model with lowest dimension. Results of the method will be shown, applied to the following problems: proper orthogonal decomposition (POD), active subspaces [2], and low-rank matrix completion.