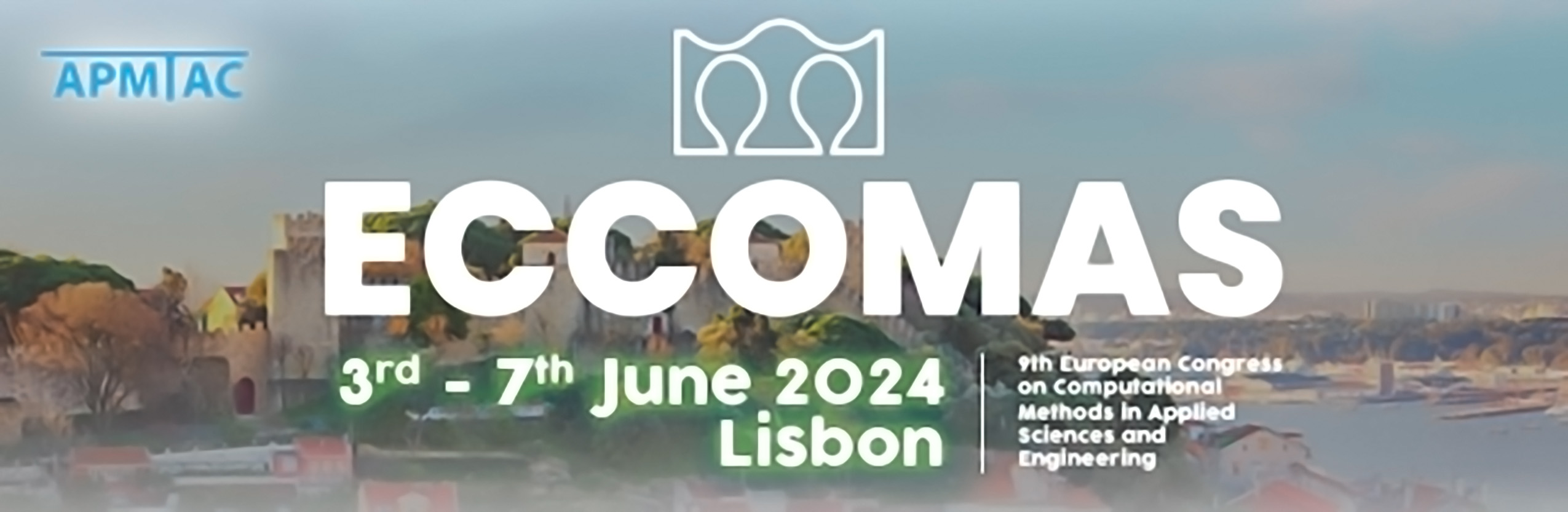
Parametric Physics-Informed Neural Networks for Material Model Calibration in Structural Health Monitoring
Please login to view abstract download link
The identification of material parameters occurring in constitutive equations is essential in structural health monitoring (SHM) as they directly reflect the resistance of the structure to external impacts and indicate possible damage and material degradation. We applied a physics-informed neural network (PINN) [1] for the calibration of the linear-elastic material model from full-field displacement data measured by digital image correlation (DIC). In an offline-phase, the PINN is trained to learn a parameterized solution of the underlying parametric partial differential equation without the need for training data [2]. We demonstrate the ability of the parametric PINN to act as a surrogate in a least-squares based calibration. In order to quantify the uncertainty, we further use the parametric PINN with Markov Chain Monte Carlo (MCMC) based Bayesian inference. Even with artificially noisy data, the calibration produces good results. Especially in iterative and sampling based methods, parametric PINNs have the advantage that model evaluation is very cheap compared to, e.g., the finite element method. Moreover, PINNs use a continuous ansatz and thereby avoid the need to interpolate between sensor and simulation domain and vice versa. In addition, we apply the parametric PINN to real experimental DIC data and investigate the extension to more complex material models. Furthermore, following the statistical model proposed in [3] , we plan to quantify the model inadequacy and consider it as another source of uncertainty. References: [1] M. Raissi, P. Perdikaris and G. E. Karniadakis, Physics-informed neural networks: A deep learning framework for solving forward and inverse problems involving nonlinear partial differential equations. J. Comput. Phys., Vol. 378, pp. 686-707, 2019. [2] E. Hosseini, P. G. Ghanbari, O. Müller, R. Molinaro and S. Mishra, Single-track thermal analysis of laser powder bed fusion process: Parametric solution through physics-informed neural networks. Comput. Methods Appl. Mech. Engrg., Vol. 410, pp. 116019, 2023. [3] M. C. Kennedy and A. O'Hagan, Bayesian calibration of computer models. J. R. Statist. Soc. B, Vol. 63, Part 3, pp. 425-464, 2001.