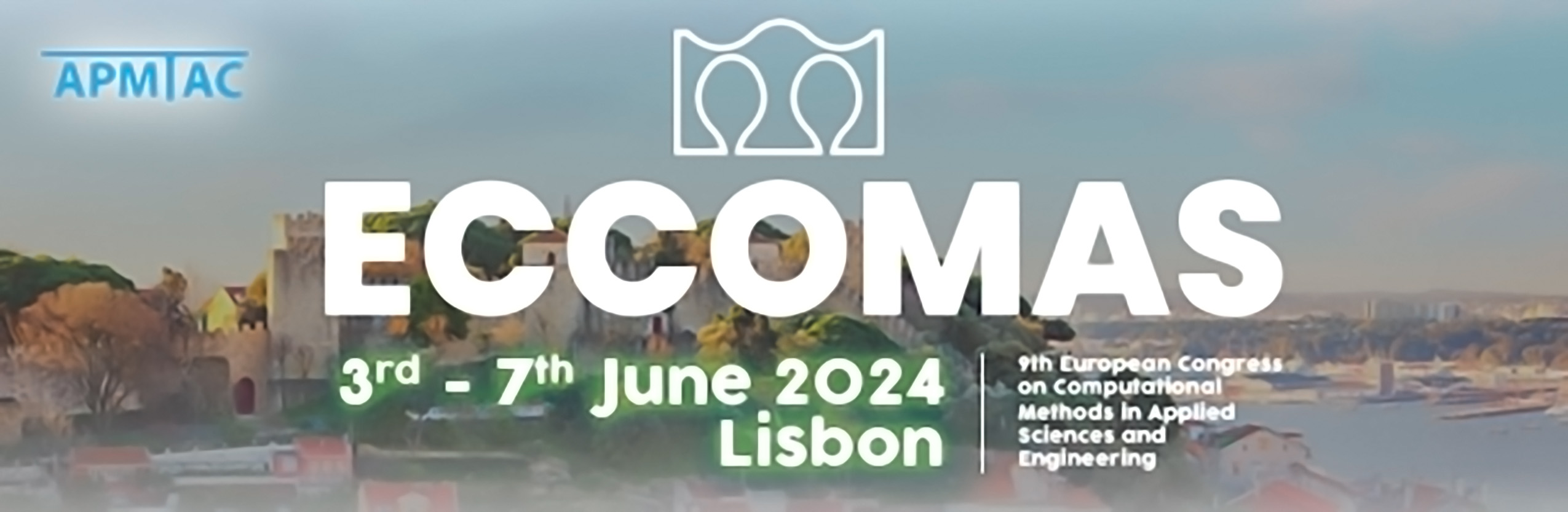
GNN accelerated Simulation of Water Flow in the Windshield Wiper System
Please login to view abstract download link
Traditional numerical methods pose significant computational challenges when simulating water flow in the windshield wiper system, making them impractical for large-scale parameter studies in this area. This shortcoming makes this problem a good candidate for a machine learning approach, which is known to have the potential to lead to a speed-up compared to traditional numerical methods. There is no specific mention of machine learning based simulations for windshield wipers in the literature so far. Addressing this need, we develop a machine learning model using a Graph Neural Network (GNN) trained on Smoothed Particle Hydrodynamics (SPH) simulations. SPH is capable of modeling the flow on a free surface and the interaction between fluid and structure, thus meeting the physical challenges of numerical modeling. The SPH simulations are carried out for cases that are less extensive and complex than the actual problem in order to keep the computational effort to a minimum. While SPH accurately models the underlying physics, our GNN generalizes these principles to unseen, geometrically complex and large scenarios of water flow on windshields interacting with a wiper, without the time-step limitation of classical numerical methods. Our approach achieves good consistency compared to a pure SPH approach, but with a significantly reduced simulation time. This shows that our machine learning model is a convincing solution for efficient parameter studies in the field of automotive water management.