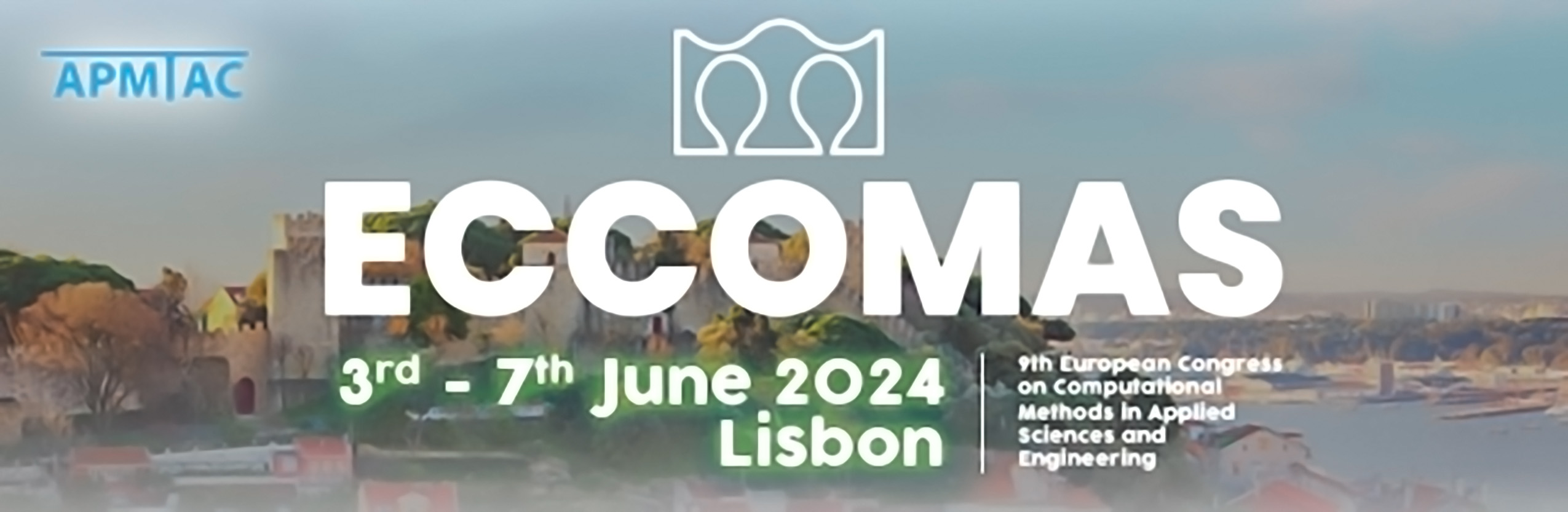
Sampling and Estimating the Set of Pareto Optimal Solutions in Stochastic Multi-Objective Optimization.
Please login to view abstract download link
Including uncertainty sources in multi-objective optimization allows more robust design decisions at the cost of dealing with stochastic quantities of interest. The objectives are then classically defined as the expectations of random quantities of interest, and their estimation can be computationally expensive. Robbins-Monro-type algorithms are an attractive alternative in this context, allowing for the minimization of the objectives through noisy gradient updates. The stochastic multi-gradient algorithm (SMGDA)[1] extends the Robbins-Monro approach to the multi-objective case. However, a bias in the algorithm and the inherent noise in stochastic gradients cause the algorithm to converge to only a subset of the whole Pareto front. Our contribution builds on the stochastic multi-gradient algorithm to reliably estimate the whole Pareto front (objective space) and Pareto optimal points (design space). First, we reduce the bias of the stochastic multi-gradient calculation using an exponential smoothing technique. Second, we simultaneously remove the remaining bias and promote the exploration of the Pareto front by adding non-vanishing noise tangential to the front. We prove that this algorithm generates samples in a concentrated set containing the whole Pareto front. Finally, we estimate the set of Pareto optimal design points using only the sequence generated during optimization. We also provide bootstrapped confidence intervals using a nearest-neighbor model calibrated with a novel procedure based on the hypervolume metric. Our proposed method allows for the estimation of the whole of the Pareto front using significantly fewer evaluations of the random quantities of interest when compared to a direct sample-based estimation, which is valuable in the context of costly model evaluations. We illustrate the efficacy of our approach with numerical examples in increasing dimension and discuss how to apply the method to more complex problems. References [1] F. Poirion Q. Mercier and J.-A. D ́esid ́eri. “SMGDA: an uncertainty based multi- objective optimization approach. Illustration to an airplane composite material”. In: Procedia engineering 199 (2017), pp. 1199–1203