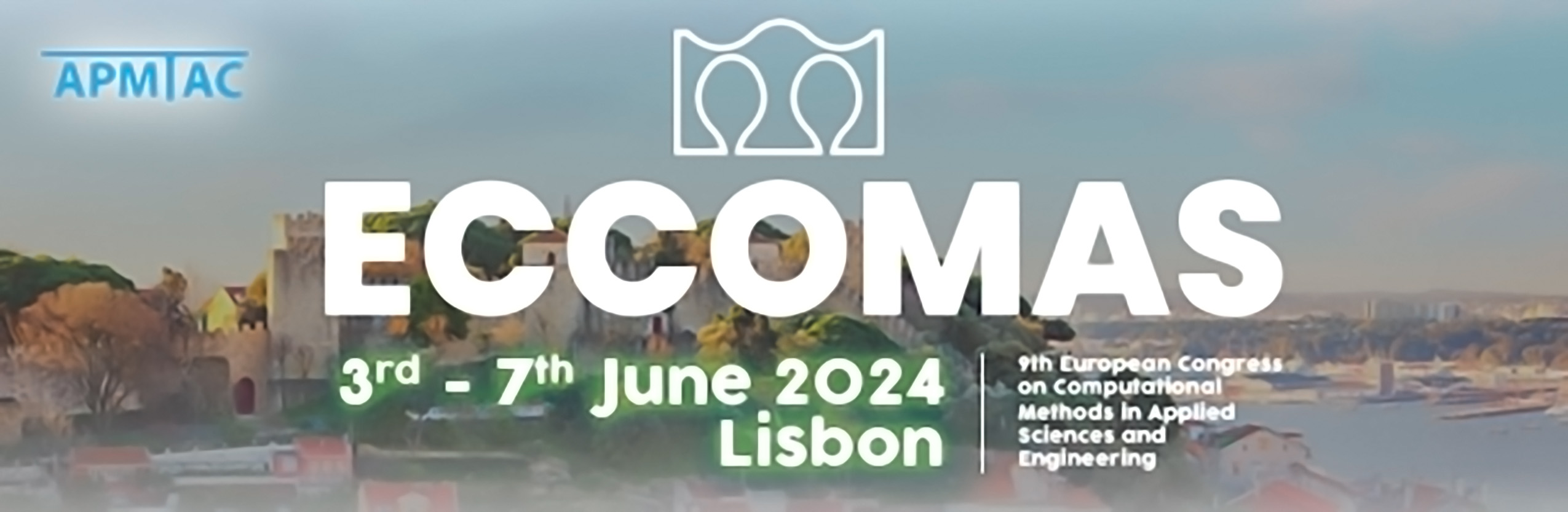
Entropy-regularized Wasserstein distributionally robust shape and topology optimization
Please login to view abstract download link
In this presentation, we explore the application of the recent paradigm of distributional robustness in the field of shape and topology optimization. Acknowledging that the probability law of uncertain physical data is rarely known beyond a rough approximation constructed from observed samples, we optimize the worst-case value of the expected cost of a design when the probability law of the uncertainty is close'' to the estimated one up to a prescribed threshold. The "proximity'' between probability laws is quantified by the Wasserstein distance, attached to optimal transport theory. The classical entropic regularization technique in this field combined with recent results from convex duality theory allow to reformulate the distributionally robust optimization problem in a way which is tractable for computations. Several numerical examples are presented, in the different settings of density-based topology optimization and geometric shape optimization. They exemplify the relevance and applicability of the proposed formulation regardless of the retained optimal design framework.