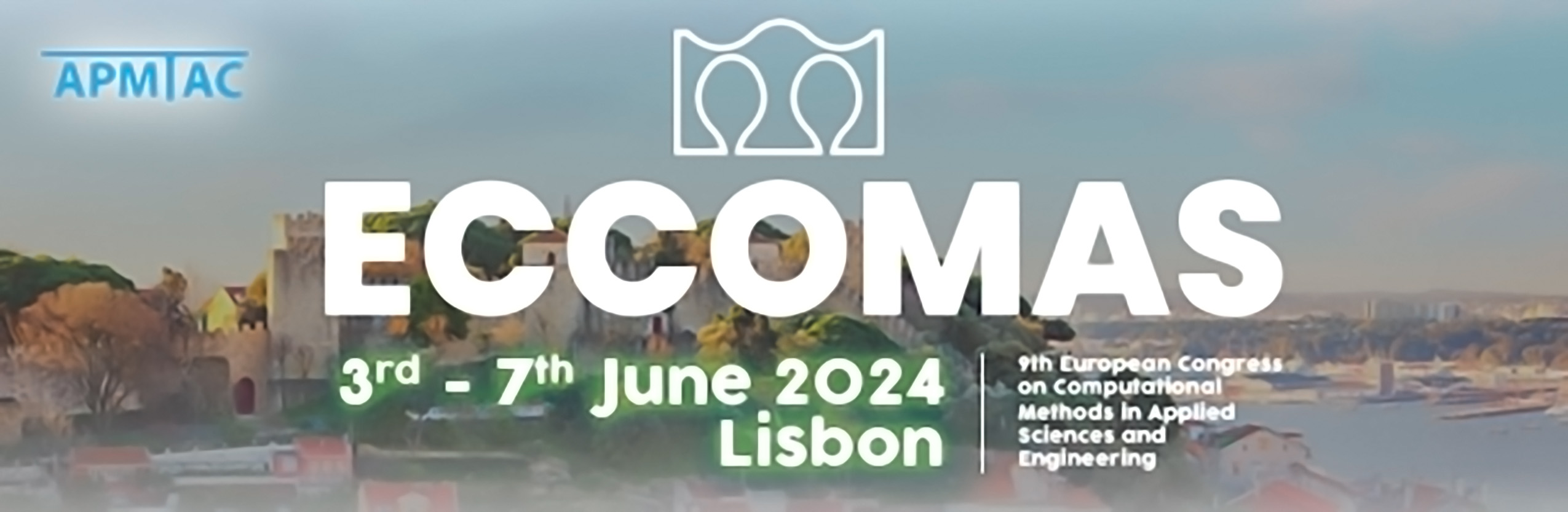
Data-Driven Calibration of RANS Transition Models with Deep Learning
Please login to view abstract download link
This study introduces a novel framework that harnesses the capabilities of deep neural networks to optimize the coefficients of a transition model, ensuring alignment with existing experimental data. Our approach has been validated using the widely recognized Gamma transition model developed by Menter et al. [1] and the comprehensive ERCOFTAC T3 flat plate experiment series [2]. The process entails the construction of an extensive database by adjusting the transition model coefficients, which then informs a fully connected neural network designed to predict wall shear stress. After training, the neural network tackles the inverse problem of identifying the coefficient set that yields the closest match to experimental wall shear stress measurements. The application of our method to a zero-pressure gradient transitional flat plate resulted in an accurate prediction of the transition location. However, achieving a precise match for the entire wall shear stress distribution was challenged by factors not included as neural network inputs, such as freestream velocity, turbulence intensity, and turbulence viscosity ratio. Our results not only demonstrate the potential of our framework, but also establish a foundation for a tool that could revolutionize how researchers and industry professionals automatically calibrate transition model coefficients, a process traditionally characterized by significant manual intervention.