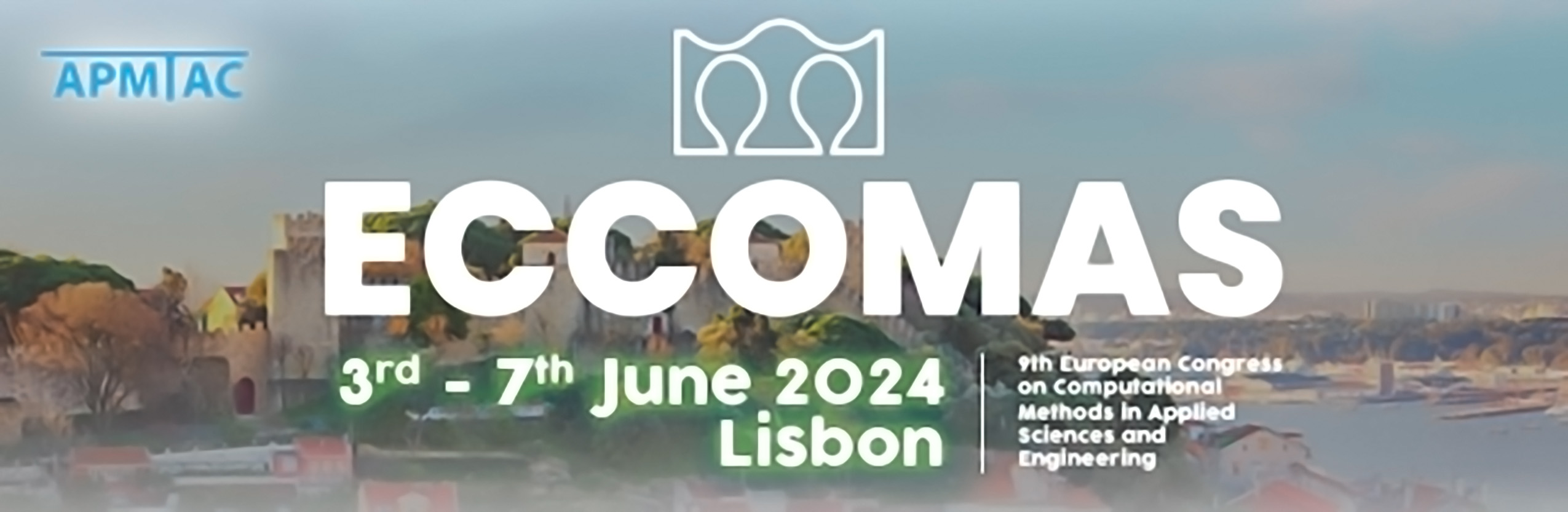
MS173 - Explainable AI for Computational Mechanics
Keywords: Algorithm Design, Anomaly Detection , Computational Fluid Mechanics, Computational Solid Mechanics, Interpretable Machine Learning, Sensitivity Analysis, Uncertainty Quantification, Variable Screening, Explainable AI
The mini-symposium “Explainable AI for Computational Mechanics†will bring together researchers from academia and industry working on the development of efficient techniques that are suitable for enhancing the transparency, accountability, and understanding of Artificial Intelligence (AI) methods when applied in Computational Mechanics.
Computational Mechanics plays a crucial role in the design, analysis, and optimization of complex mechanical systems, where precise predictions and decisions are essential for ensuring safety and efficiency in real-world situations. However, merely providing inference is no longer sufficient. Thus, Explainable AI (XAI) is getting an increasingly important role in Computational Mechanics. Before deploying AI methods, domain experts and engineers seek answers to questions such as how a machine learning model works, what parameters play a role in the prediction and what uncertainties and biases are incorporated in the data and the models.
Our mini-symposium welcomes contributions aimed at providing innovative perspectives on the usage of XAI in Computational Mechanics tasks related to the study of structures, materials, and fluids. We will consider both applied and methodological studies, offering valuable insights into (1) concrete results of using XAI to make better-informed decisions in engineering design problems, and (2) interesting XAI methods that show high application potential in real-world situations, including the field of Computational Mechanics.
Contributions may address new developments in the following subfields (non-exclusive list):
• Model prediction interpretation
• Feature Importance and Sensitivity Analysis
• Uncertainty Quantification
• Algorithm Design and Model Selection
• Human-centered Design
• Anomaly Detection
We look forward to receiving your contribution!