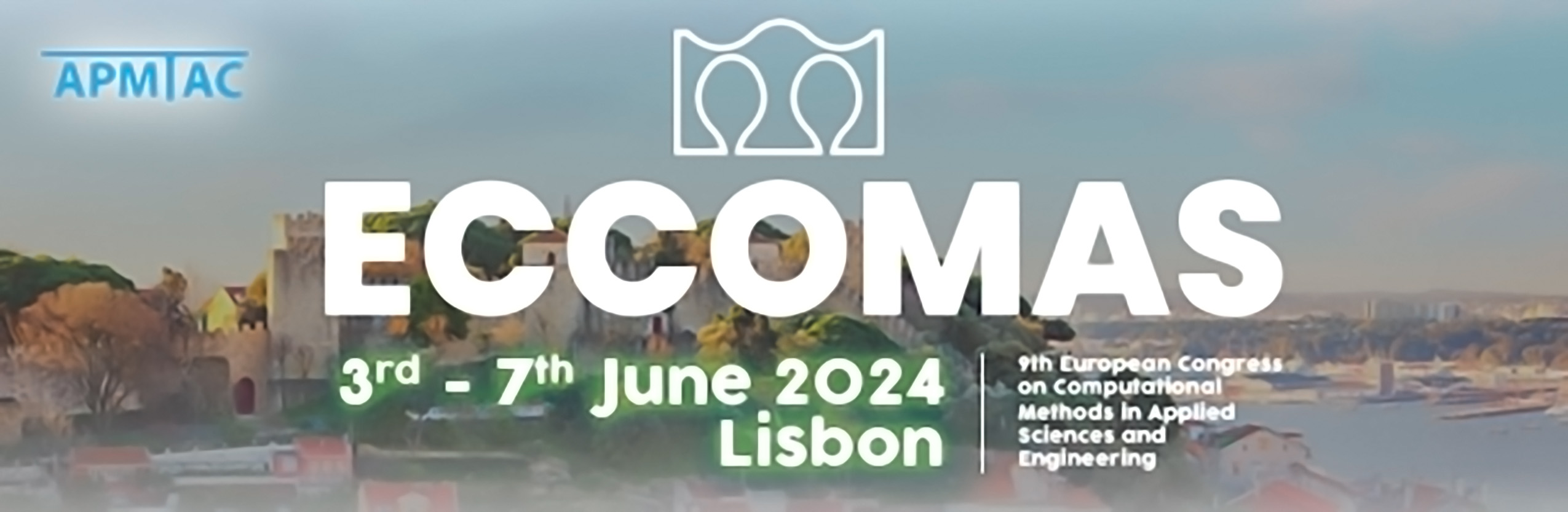
MS166 - Advancing SCiML Surrogates via Numerical Methods and Vice Versa
Keywords: Hybrid Methods, numerical methods, Scientific Machine Learning
The field of scientific machine learning (SciML) deals with the combination of scientific computing and machine learning. A major impact of SciML on computational modeling has been made by the development of efficient surrogates that expand the capabilities of traditional numerical methods by using data-driven models. However, data-driven surrogates also encounter challenges, including the computationally intensive training and the lack of explicit error control and robustness, which limit their reliability in real-world applications.
In this mini-symposium, we will discuss recent developments on the enhancement of data-driven SciML surrogates via numerical methods, and vice versa, to combine the strengths and alleviate weaknesses. We invite submissions on various topics concerning the integration of deep learning and classical numerical methods , encompassing, but not limited to:
• The development of novel types of SciML surrogates, including approaches that incorporate physical and geometric constraints into the architectural design of neural networks.
• The integration of state-of-the-art numerical methods with SciML techniques to enhance the training process.
• Techniques that combine SciML surrogates with state-of-the-art numerical solution methods for increased efficiency and efficacy.
• Hybridization of standard numerical methods (Finite Element, Iso-geometric analysis, etc.) with deep learning algorithms.