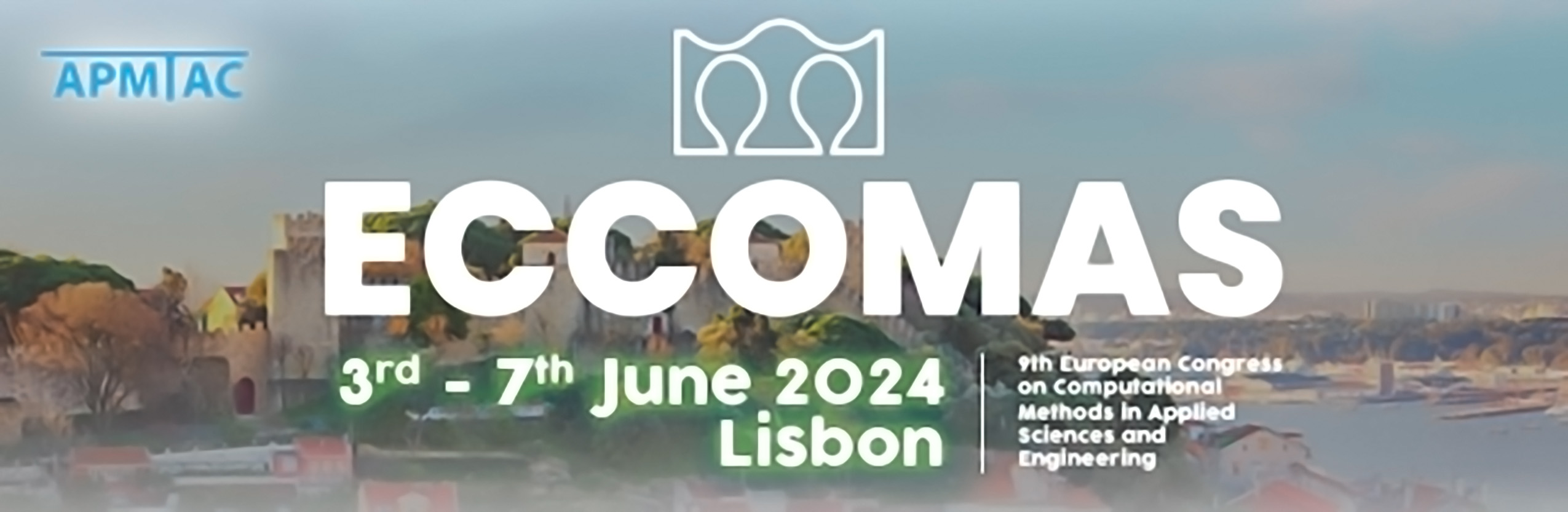
MS123 - Bayesian Inversion for model calibration and digital twinning in engineering
Keywords: Bayesian Inference, Digital Twins, inverse problems, Machine learning, model updating, probabilistic optimization, Surrogate Modelling
Computational models play a crucial role in predicting the behavior of complex physical and mechanical systems. However, the parameters within these models are often influenced by uncertainties, making the determination of the model responses troublesome. Bayesian inference emerges as a potent statistical tool for inferring these uncertain model parameters using available data and information. To assure that the numerical model is trustworthy enough, one must take into account the prior uncertainties of these input parameters or fields, and improve these models by data (e.g. of model responses) gained from some higher fidelity numerical model or from physical experiments, or even by the observation of the actual system.
The problems of uncertainty quantification and Bayesian updating have gained significant attention in the field of computational science and engineering due to their potential to enhance decision-making, optimize system performance, and provide valuable insights into complex phenomena. Bayesian inference methods typically rely on sampling algorithms. When dealing with computationally expensive models, such exploration demands substantial computational resources. As a result, recent research endeavors concentrate on developing efficient Bayesian inference methods through surrogate modelling and novel mathematical formulations and advanced sampling and filtering techniques. Methods facilitating a sequential update of the model parameters based on continuously observed responses have also enabled the “digital twinning†concept. This chimes very well with the view that machine learning is in fact a Bayesian estimate of a conditional expectation; and as such machine learning procedures for digital twins are also in the scope of the mini-symposium.
This mini-symposium serves as a platform to discuss the latest developments and foster collaboration among researchers working on Bayesian inference. The scope encompasses a range of topics, including but not limited to methodological advancements or novel applications, advanced methods for Bayesian analysis, machine learning, inference techniques accounting for spatial or temporal dependence of uncertainty, recursive or online Bayesian inference, hierarchical models, optimal experimental design, Bayesian inference utilizing surrogate models, accelerated Bayesian inference leveraging machine learning, and high-performance computing.