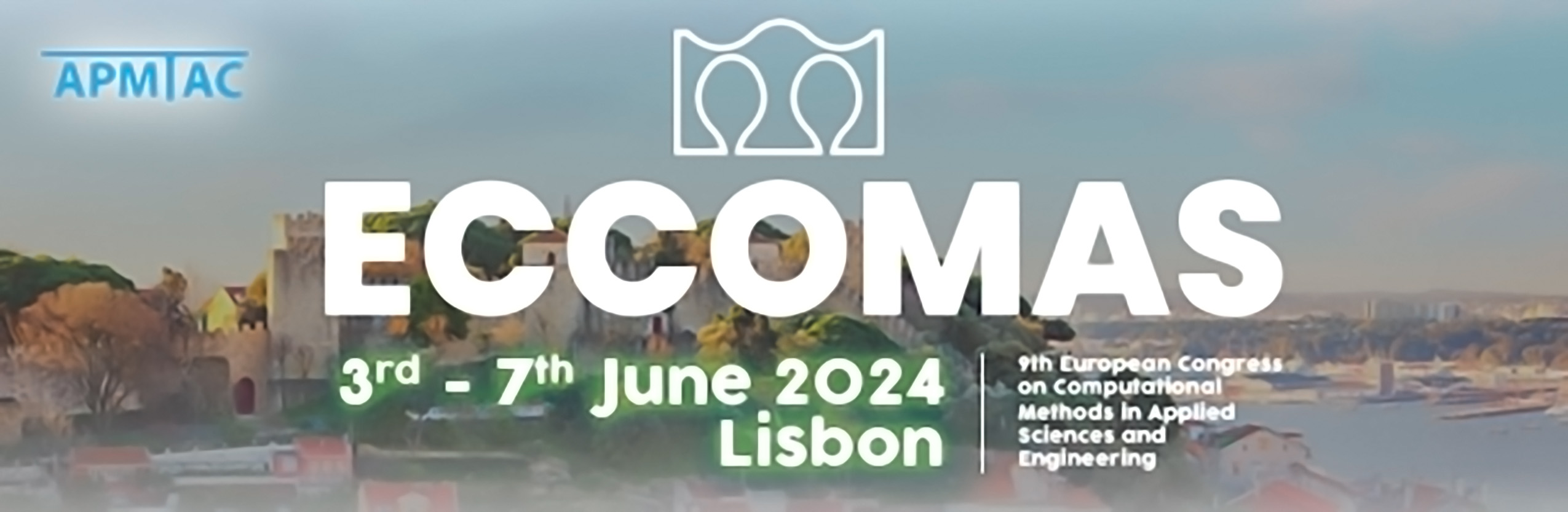
MS103 - Computational Challenges in Industry and Sustainable Development: Sampling, Surrogate and Multi-fidelity Models for Inverse Analysis, Uncertainty Quantification and Optimisation
Keywords: Digital Twins, environmental application, industry, inverse problems, mathematics of planet earth, multi-fidelity, Optimisation, sampling, Scientific Machine Learning, smart cities, surrogate models, sustainable development, uncertainty quantification
The conception, design and operation of complex systems entail computationally-intensive procedures, such as inverse problems, uncertainty quantification, data assimilation, real-time monitoring and optimisation.
Efficient sampling and surrogate modelling techniques, as well as multi-fidelity approaches, are among the key enabling technologies to make such problems treatable. These techniques should not be understood as mutually alternative options, but rather as complementary tools. For instance, surrogate models allow for substantial speed-up of computations but are crucially grounded on the ability to efficiently sample the parameter space. Moreover, when sampling expensive models, multi-fidelity approaches become essential, since they allow to appropriately allocate the computational effort on models with different degrees of accuracy, depending on the specific needs of the target application and on the region of the parameter space under investigation (exploration vs. exploitation).
This minisymposium aims to gather contributions on state-of-the-art techniques in scientific machine learning (understood in a broad sense, from reduced-order modelling and statistical computing to deep and reinforcement learning) and to foster the cross-dissemination of ideas towards the development of multi-fidelity and multi-disciplinary digital twins. Particular attention will be devoted to the challenges of blending experimental data, physical models and mechanistic knowledge of different fidelities. This is especially critical in the context of data-driven engineering, where information stemming from different sources, with different cost and reliability, is available. Application-oriented contributions tackling problems related to industry, sustainability, smart cities and environmental issues are particularly welcome.