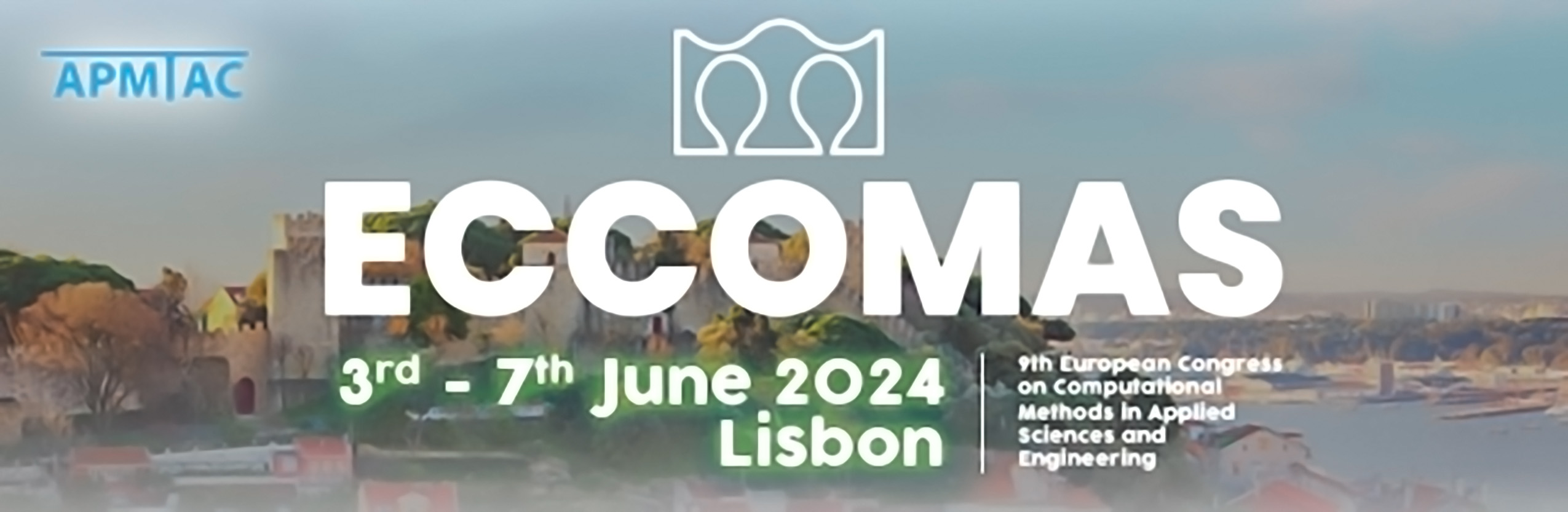
MS094 - Advances in UQ and Data-driven Methods for Scale-resolving Turbulence Simulations
Keywords: Data-driven models, Machine learning , Multi-fidelity models, Uncertainty quantification (UQ), Turbulence simulation
High-fidelity scale-resolving simulations of turbulent flows have an utmost importance for understanding the flow physics and achieving optimal engineering designs. Such simulation approaches which include DNS, LES, and hybrid RANS-LES [1] require (prohibitively) large computational resources. Moreover, their resulting quantities of interest are uncertain up to some extent due to various sources. Therefore, not only the accurate quantification of uncertainties for such simulations is vital, but also cost-effective techniques must be considered when addressing outer-loop problems where several flow realisations are required.
This minisymposium aims at gathering experts in the theoretical development and application of uncertainty quantification (UQ) and data-driven approaches for scale-resolving simulations of turbulent flows. The topics of interest include, but are not limited to, forward and inverse UQ problems, error estimation, Bayesian optimization, multi-fidelity/multi-level models, sensitivity analysis, predictive machine learning models, reduced-order and surrogate models [2]. A particular focus will be on the strategies capable of making the overall computational cost of the data-driven methods affordable while retaining high accuracy.