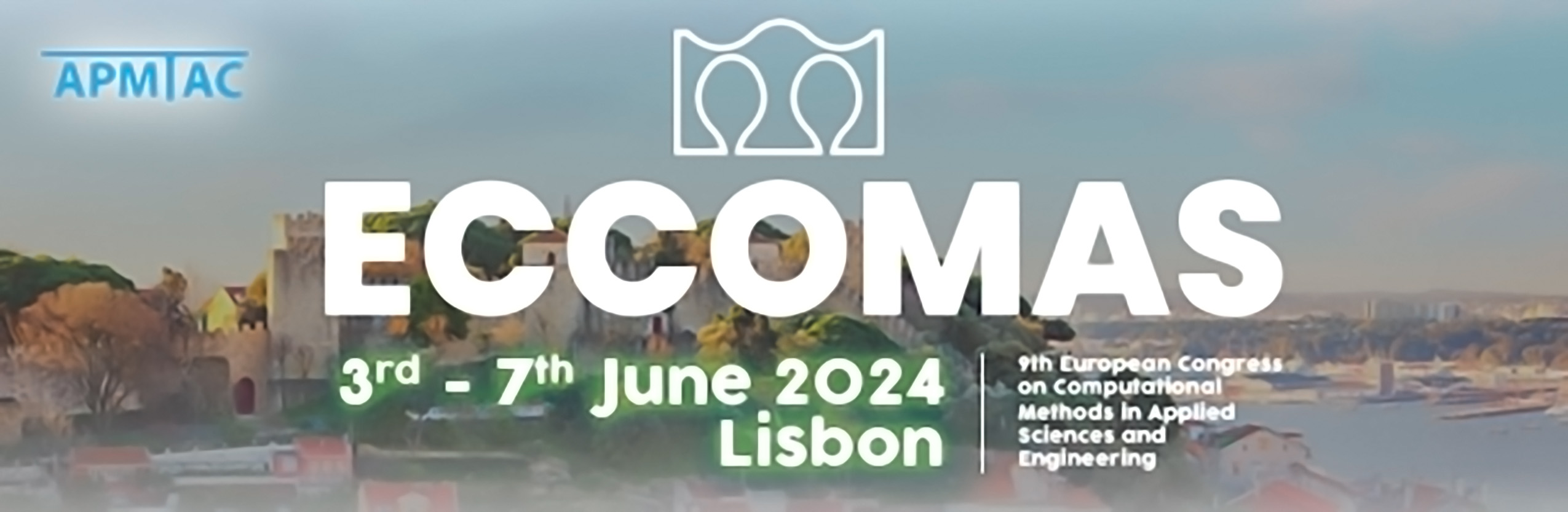
MS092 - Machine Learning-based Latent-Space Models for Multiscale Simulations
Keywords: Multi-scale modelling, Scientific Machine Learning
Multiscale problems are inherently high-dimensional and typically require (excessively) high resolution discretizations. This poses major computational problems and often renders the problem infeasible to solve within a satisfying time frame. To alleviate these problems, some form of dimensionality reduction is required. Often the larger scales in the problem are still computed directly (for instance by filtering the governing equations), yet the effect of the small scales can only be approximated by so-called closure models or empirical constitutive equations. While traditionally these were based on approximate physical models, current research focuses on machine-learning (ML) based closure models which could offer better accuracy.
In the machine learning community so-called latent-space models are an active area of research. The general idea is that most problems, while posed in a high-dimensional space, actually lie on a low-dimensional manifold. The task at hand is then to identify an optimal latent representation. In this regard, deep learning has shown to be an excellent framework due the capabilities of handling high-dimensional and non-linear problems. In this mini symposium will we focus on the application ML-based latent space methods to the problem of multiscale (closure) modelling. There are still many open questions to be answered. For instance, how do we know when we have an optimal latent-space representation? Should the latent-space ML model be deterministic or stochastic? How do we ensure that the coupling between latent-space ML model and the large-scale physical model remains stable? How do we efficiently incorporate information from the high-fidelity space into the latent space? We welcome talks from both a theoretical and a broad multiscale application perspective.