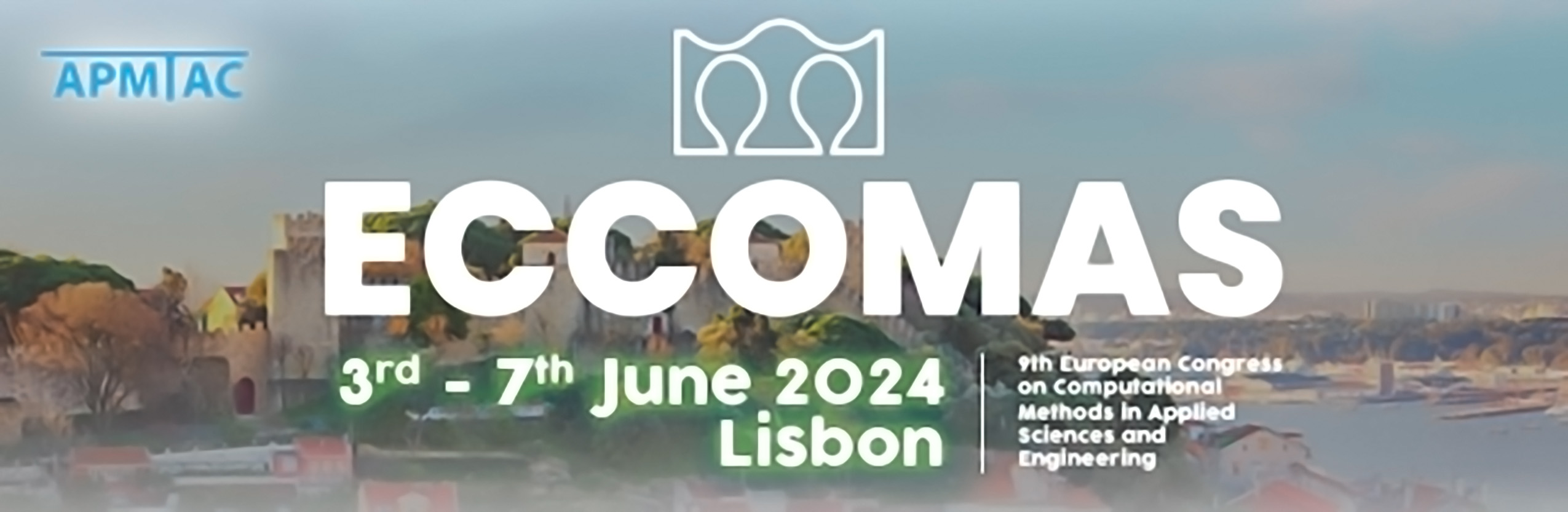
MS081 - Gradient-Based and Gradient-Free Optimization Methods, with emphasis on Innovative Designs for a Climate Neutral Aviation
Keywords: adjoint, gradient-based, gradient-free, machine learning, multi-disciplinary optimization, robust optimization
This MS is in the area of gradient-free and gradient-based (mostly discrete and continuous adjoint) optimization methods as primarily used in fluid and solid mechanics. Support by reduced order models and Machine Learning techniques for these kinds of applications is also in the scope of the MS. Papers in either Single- or, particularly, Multi-Objective Optimization, including also design under uncertainties (and, thus, uncertainty quantification) are welcome. Emphasis is laid on the use of the above methods in the optimal/innovative designs for a climate neutral aviation. However, papers in other areas that make use of similar tools are welcome.
The topic of climate neutral aircraft has been selected as the key application area since sustain air traffic growth while reducing the environmental footprint produced by CO2, NOX emissions and aviation-induced cloudiness (such as contrails) represents a crucial challenge for the evolution of the aeronautical sector. To reach this very ambitious objective, new technologies must be developed, matured, and integrated into disruptive aircraft architectures. The development of innovative fast design tools and optimization methods for aerodynamics, structure and propulsion is therefore crucial to this goal. Unlike conventional aircraft architectures and engine systems, for which an exhaustive return on experience is available, the design of breakthrough technologies and new concepts requires enhanced simulation approaches, able to easily integrate high fidelity modelling, ingest real data as well as to account for tighter multi-physics couplings and uncertainties. Although advanced simulation models can account for epistemic uncertainty (through full resolved physics and data assimilation), additional sources of uncertainty are unavoidably introduced by manufacturing processes and the variability of operating conditions across the lifespan (also due to progressive deterioration or damage). Therefore the development of more efficient robust optimization capabilities is of utmost importance to avert showstoppers and reduce all potential barriers to the entry into service of future green aircraft and engine.