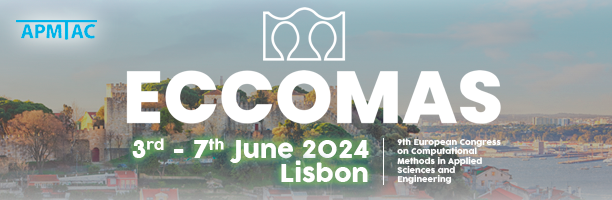
MS075 - Recent Advances in Deep Reinforcement Learning of Complex Dynamical Systems
Keywords: Deep reinforcement learning, optimal control, PDEs
ABSTRACT
Optimal control of dynamical systems has become a crucial task in the scientific and engineering community. Some examples are related to drag and turbulence reduction, efficient heat transfer, and shape optimization. Many of these systems are described by high-dimensional, nonlinear, and time-dependent partial differential equations (PDEs). The complexity of these systems hinders the use of traditional optimal control methods, such as, e.g., the Hamilton-Jacobi-Bellman equation or the adjoint method, due to poor scaling properties with respect to the problem dimensionality.
Deep learning has emerged as a powerful tool for tackling the challenges of reduce order modelling, and operator inference for high-dimensional, and complex PDEs. However, only in recent years, data-driven approaches have been applied to optimal control of PDEs. In particular, deep reinforcement learning (DRL) results to be a way to perform scalable optimal control from measurements. DRL is a mathematical framework for solving sequential decision-making problems from the interaction of the agent, i.e., the decision maker, and its environment. DRL has achieved outstanding success in different fields such as video games, robotics, finance, and even health applications. However, despite the success, DRL has only recently been employed to control complex PDEs leaving a vast gap in the literature [1].
This minisymposium will gather a broad spectrum of contributions in the very active and recent research field of DRL for optimal control strategies discovery, emphasizing theoretical aspects, computational performance, and practical examples in scientific applications.
REFERENCES
[1] Colin, V., Rabault, J. and Vinuesa, R. “Recent advances in applying deep reinforcement learning for flow control: Perspectives and future directions†Physics of Fluids 35.3 (2023).
[2] Botteghi, N. et al. “Low dimensional state representation learning with robotics priors in continuous action spaces†2021 IEEE/RSJ International Conference on Intelligent Robots and Systems (IROS) (2021).