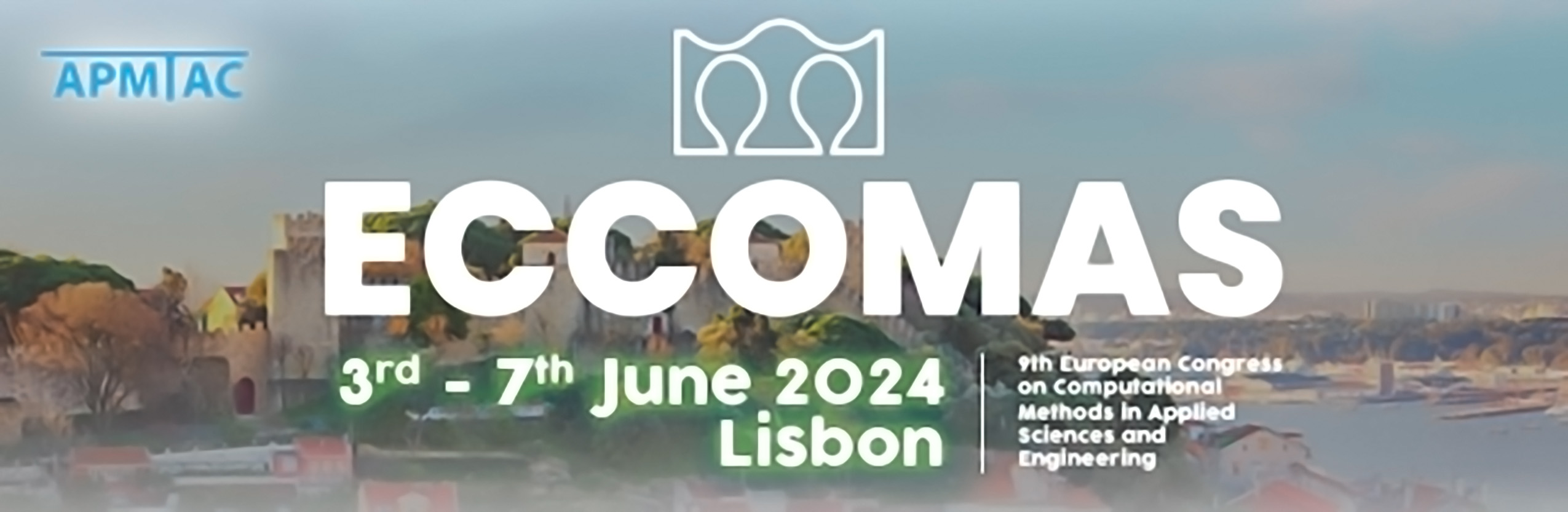
MS073 - Towards Digital Twins for Infrastructures
Keywords: Data-driven Models, Infrastructure, Physical Models, Digital Twins, Experimental Data Sources, Sensors as Data Source
Digital twins are a powerful tool to design, optimize, monitor, operate and service real (physi-cal) objects by allowing for holistic and realistic simulations and predictions. The digital repre-sentation of a real object combines all relevant and available models, data and information about its real counterpart. Thereby, the coexisting digital and real twin are able to exchange information bi-directionally [1]. While the term digital twin arose in the context of manufac-turing (Industry 4.0), the concept is more and more explored in various other fields such as health care, education, meteorology and construction, too [2]. Using the great potential of digital twins for various and critical infrastructures, like road systems, bridges, water treat-ment facilities and energy networks, which are valuable and expensive goods of our society, is meaningful to increase among others safety, sustainability and operability. However, the de-velopment of digital twins at hand of sub-models, data and interfaces requires huge interdis-ciplinary knowledge and contributions on, e.g., coupling of models, domain knowledge, so-phisticated data science and machine learning.
The objective of our mini-symposium is to bring experts in the field of digital twins for infra-structures and their enabling technologies together to increase the interdisciplinary knowledge and to foster scientific exchange and collaboration.
Topics of interest include, but are not limited to:
• efficient physical and data-driven models,
• obtaining and processing of sensor data from real objects and experiments as data source for digital twins,
• scientific machine learning for digital twins, e.g. physics-informed neural networks,
• approaches to combine different models and data of a real object in one digital repre-sentation,
• twinning approaches to keep the real object and its digital representation consistent,
• architectures and use cases of digital twins,
• treatment of uncertainties within digital twins.
REFERENCES
[1] M. Asch, A Toolbox for Digital Twins: From Model-Based to Data-Driven, Philadelphia, PA: Society for Industrial and Applied Mathematics, 2022.
[2] A. Rasheed, O. San and T. Kvamsdal, “Digital Twin: Values, Challenges and Enablers From a Modeling Perspectiveâ€, IEEE Access, Vol. 8, pp. 21980ï€22012, (2020).