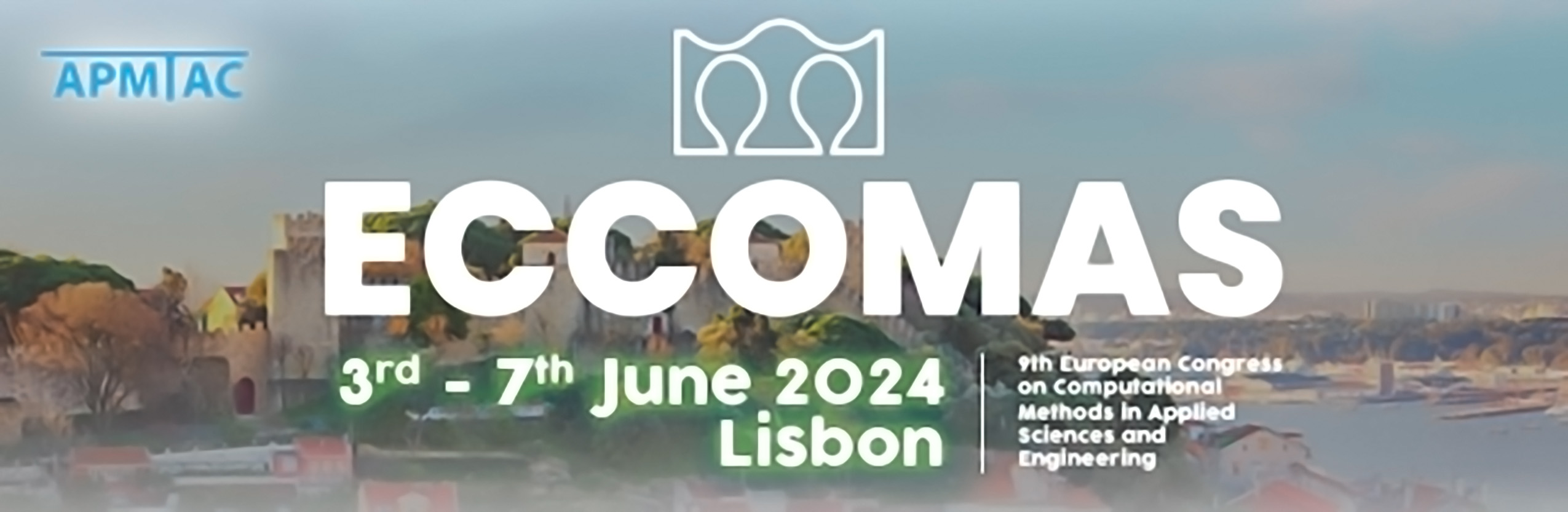
MS052 - Efficient SciML Techniques With Applications to CFD
Keywords: Computational Fluid Dynamics, Domain Decomposition, Multilevel, Partitioning, Scalable Machine Learning, Multiscale models, Scientific Machine Learning
Scientific machine learning (SciML) has emerged as a transformative field at the intersection of scientific computing and machine learning. This mini-symposium aims to explore the latest advancements on efficient SciML algorithms and their application to computational fluid dynamics (CFD) problems. This includes (but is not restricted to):
+ Neural network-based reduced order models for (high-dimensional) parametrized and inverse problems
+ Neural network-augmented numerical schemes for CFD problems
+ Multilevel and multiscale machine learning models
+ Scalable machine learning algorithms based on (space-time) domain decomposition + Data- and model-based parallelization of machine learning algorithms
CFD applications may range from model problems of simple linear flow models to highly nonlinear multiphase and multiscale flows as well as multiphysics problems which include a fluid component from real-world applications.