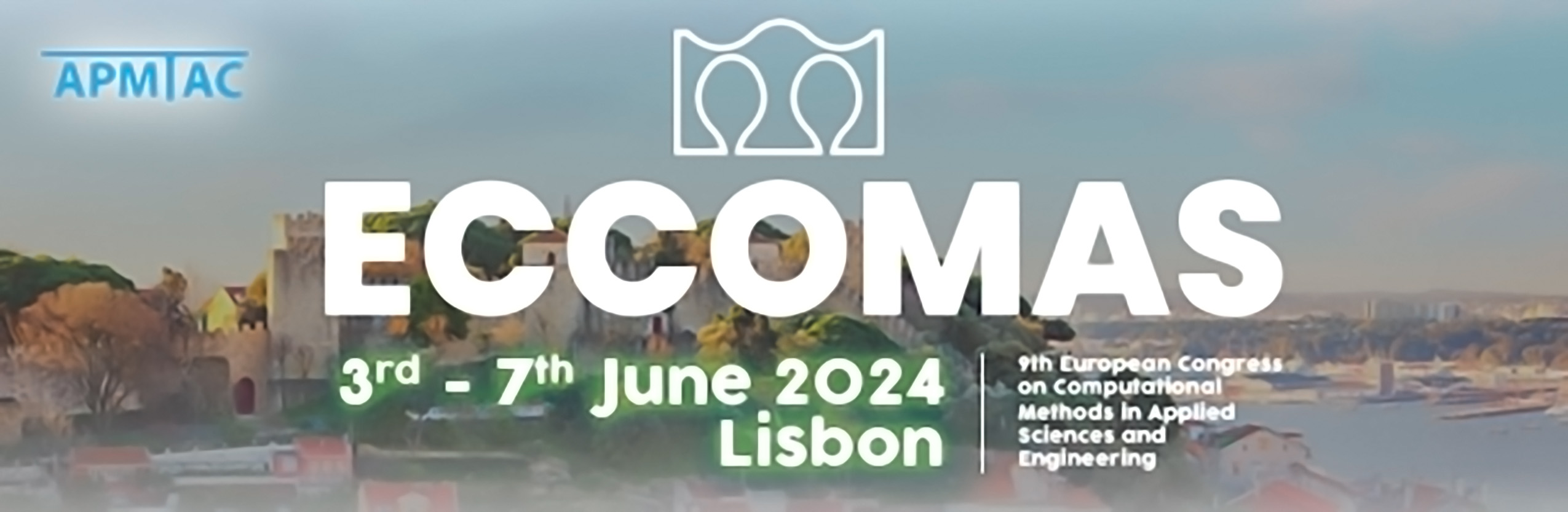
MS035 - Computational Models and Methods for Predicting Cancer Progression and Treatment Response
Keywords: cancer, cancer forecasting, computational oncology, digital twins, inverse problems, machine learning, mechanistic modeling, model selection, optimal control theory, uncertainty quantification
Cancers are highly heterogeneous diseases that involve diverse biological mechanisms, interacting and evolving at various spatial and temporal scales. Multiple experimental, histopathological, clinical, and imaging methods provide a means to characterize the heterogeneous and multiscale nature of these diseases by providing a wealth of temporally and spatially resolved data on their development and response to therapies (e.g., cancer architecture, mechanics, and vascularity; cancer cell mobility and proliferation; drug transport and effects). These multimodal, multiscale datasets can be exploited to constrain biophysical models of cancer growth and treatment response both in preclinical and clinical settings. These models can then be leveraged to test hypotheses, produce individualized cancer forecasts to guide clinical decision-making, and, ultimately, to design optimized therapies. The overall goal of this minisymposium is to provide a forum to present and discuss recent developments in data-informed computational models and methods for predicting cancer growth and treatment response, with special focus on the following research areas: (i) biology-based mechanistic models of cancer growth and treatment in vitro and in vivo; (ii) computational methods for model initialization, parameterization, and patient-specific simulation; (iii) model-oriented, personalized optimization of treatment regimens; (iv) uncertainty quantification and model selection methods; (v) hybrid strategies combining machine learning and mechanistic modelling; and (vi) digital twins in clinical oncology.
REFERENCES
[1] G. Lorenzo, D.A: Hormuth II, A.M. Jarrett, et al., “Quantitative In Vivo Imaging to Enable Tumour Forecasting and Treatment Optimizationâ€. In: Balaz, I., Adamatzky, A. (eds), Cancer, Complexity, Computation, 46, 55-97, Springer Cham, (2022).
[2] M. Alber, A. Buganza Tepole, W.R. Cannon, et al., “Integrating machine learning and multiscale modelling - perspectives, challenges, and opportunities in the biological, biomedical, and behavioral sciencesâ€, npj Digit. Med., 2, 115, (2019).